Can China Grow Its Own AI Tech Base?
Despite market success, Chinese experts see stubborn dependencies
Blog Post
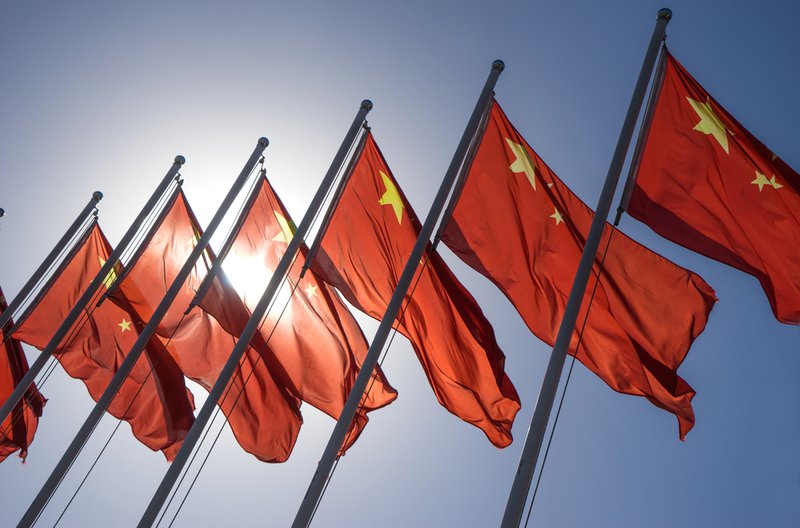
Shutterstock
Nov. 4, 2019
This article was published as part of the Stanford-New America DigiChina Project's first special report, AI Policy and China: Realities of State-Led Development. Read this article and the others in PDF here.
Last December, China’s top AI scientists gathered in Suzhou for the annual Wu Wenjun AI Science and Technology Award ceremony. They had every reason to expect a feel-good appreciation of China’s accomplishments in AI. Yet the mood was decidedly downbeat.
“After talking about our advantages, everyone mainly wants to talk about the shortcomings of Chinese AI capabilities in the near-term—where are China’s AI weaknesses,” said Li Deyi, the president of the Chinese Association for Artificial Intelligence. The main cause for concern: China’s lack of basic infrastructure for AI.
More than two years after the release of the New Generation Artificial Intelligence Development Plan (AIDP), China’s top AI experts worry that Beijing’s AI push will not live up to the hype. The concern is not just that China might be in for an “AI winter”—a cyclic downturn in AI funding and interest due to overly zealous expectations. It’s also that for all China’s strides in AI, from multi-billion dollar unicorns to a glitzy state plan, it still lacks a solid, independent base in the field’s foundational technologies.
The concern seems counterintuitive at first glance. In recent years, China has built a crop of commercial AI juggernauts with no direct counterparts elsewhere in the world. Yet, upon closer scrutiny, it’s clear that Chinese AI researchers are highly reliant on innovations and hardware built in the West.
Chinese Domestic Programming Frameworks Lag U.S. Giants
A brief glance at the infrastructure Chinese developers are using to run their algorithms reveals one reason for concern. The two dominant deep learning frameworks are TensorFlow and PyTorch, developed by Google and Facebook, respectively. A “framework” is essentially a set of programming shortcuts that makes it simpler for researchers and engineers to design, train, and experiment with AI models. Most AI research and deployment uses one framework or another, because frameworks make it possible to use common deep learning concepts (such as certain types of hidden layers or activation functions) without directly implementing the relevant math.
While Chinese alternatives to TensorFlow and PyTorch exist, they have struggled to gain ground. Baidu’s PaddlePaddle scarcely appears in either English- or Chinese-language listicles of top framework comparisons. Although it’s difficult to find reliable and up-to-date usage statistics, various informal indicators all point to a large discrepancy in usage. According to Github activity, Baidu’s PaddlePaddle trails PyTorch and TensorFlow by a factor of 3–10 on various statistics. In one Zhihu thread on comparing frameworks, only one user stood up for PaddlePaddle—the PaddlePaddle official account.
In the short term, the popularity of different frameworks may not matter much for AI research in China. But in the longer term, it’s hard to imagine China’s AI sector achieving the State Council’s ambition to reach “world-leading levels” if the foundational software underlying its own research is built in the United States. What’s more, the network effects that arise because researchers want to use the same frameworks as their collaborators (and because frameworks with more users are generally better maintained over time) mean it could be increasingly difficult for a Chinese company to come from behind and dethrone established frameworks.
No Clear Escape From GPUs or U.S.-Made Successors
When it comes to AI hardware, the outlook is equally troubling for China. Despite buzz in venture capital circles about Chinese AI chip startups like Cambricon and Horizon Robotics, Chinese AI developers continue to rely heavily on western hardware to train their neural networks. This is because Chinese AI chips have so far largely been confined to “inference,” or running existing neural network models. In order to “train” those neural nets in the first place, researchers need high-performance, specialized hardware. Unlike most computational tasks, training a neural network requires massive numbers of calculations to be performed in parallel. To accomplish this, AI researchers around the world rely heavily on graphics processing units (GPUs) that are mainly produced by U.S. semiconductor company Nvidia.
Originally designed for computer graphics, the parallel structure of GPUs has made them convenient platforms for training neural networks. SenseTime’s supercomputing center DeepLink, for instance, is built on a staggering 14,000 GPUs. However, GPUs are not the only hardware platform that can train neural nets. Several chips including Google’s Tensor Processing Unit (TPU) and field-programmable gate arrays (FPGAs) from companies like Intel and Xilinx will likely reduce the importance of Nvidia GPUs over time. Notably, none of these competitors to the GPU are Chinese.
Why are there no Chinese competitors challenging the GPU’s reign? The answer, according to Sun Yongjie, a notable tech blogger in China, is that Chinese AI chips are created for “secondary development or optimization” rather than replicating fundamental innovations. The derivative nature of Chinese AI startups came into stark relief last year when California-based Xilinx bought DeePhi Tech, a trailblazing Chinese AI chip startup. The acquisition provoked immediate indignation among netizens, many of whom argued that the Chinese government should have intervened to protect one of China’s most promising chip ventures. Upon further reflection, however, several bloggers argued government intervention would be fruitless, since DeePhi’s deep learning processors are entirely built on Xilinx FPGA frameworks. “If DeePhi Tech ever broke away from Xilinx's FPGA platform, it would be completely cut off from all sustenance,” Sun wrote.
DeePhi’s technical dependence on a western chip company is not an anomaly—it’s the industry norm. Horizon Robotics, China’s largest AI chip unicorn, often billed as the “Intel of China,” built its main AI processor architecture, the Brain Processing Unit (BPU), on top of Intel’s FPGA. Most Chinese AI companies buy the license for core components, rather than developing them internally. In some cases, according to industry insiders, AI startups have even outsourced the actual chip design to more experienced western design companies.
Breakthrough vs. Implementation
It’s an open question whether any of this matters. Does China need to develop its own foundational software and hardware in order to be an AI leader, or can it build upon the existing scaffolding of western companies? If Chinese AI researchers can effectively use TensorFlow and train models on Nvidia GPUs, does it matter whether foundational platforms are also built in China?
At least one prominent voice—venture capitalist and AI scientist Kai-Fu Lee—thinks it does not. In his recent book AI Superpowers: China, Silicon Valley, and the New World Order, Lee argues that AI has entered the “age of implementation.” The foundational breakthrough of modern AI research (deep learning) has already been made, Lee claims, so now all that matters is translating and applying that breakthrough for specific use cases. In many ways, China’s approach to AI thus far appears to be based on this premise, with companies flexing their implementation muscles to reach giant valuations and fast adoption.
But Lee’s view is far from consensus among AI researchers.
The basic algorithmic ideas behind modern deep learning systems were in place by the 1980s, but constraints on data and computation made them impractical to implement until more recently. Experiments in 2011 and 2012 kickstarted the current deep learning boom, using the method to achieve state-of-the-art results in image recognition. Like most interesting advances in AI research, the novel contributions of these experiments were neither major breakthroughs nor minor tweaks of past research—they were something in-between.
Looking forward, there are plenty of reasons to expect a steady stream of medium-sized advances to continue pushing the bounds of AI. Areas that have seen significant progress in the last year or two include image generation, increasingly complex strategy games, and—most recently—language understanding and generation. The research powering each of these advances came out of labs focused on fundamental R&D, not mere “implementation.”
A consensus in the Chinese AI community has gradually formed around the view that China needs to participate in this steady foundational progress in order to become an AI powerhouse. The catalyst for this convergence in thinking was largely external: The April 2018 addition of Chinese telecommunications company ZTE to the U.S. Commerce Department’s Entity List, which lists companies and other entities U.S. firms may not export certain items to without a special license. In a speech before the National People’s Congress Standing Committee that fall, Chinese Academy of Sciences (CAS) expert Tan Tieniu* warned that China’s AI industry could face its own ZTE moment if it did not build its own foundational technology. Far from the isolated opinion of a risk-averse government scholar, the fear resonated throughout much of China’s AI community. A post on DeepTech, a popular WeChat account for AI industry news, called TensorFlow and other U.S. open-source frameworks “traps” that could “suffocate” China’s AI development, asking: “If open-source projects can be curtailed on a whim by export bans, will China’s AI companies be next?”
Aside from the threat of U.S. export control, prominent members of China’s AI community have become more vocal about the constraints that a lack of independent foundations could place on China’s AI development. “As the AI era progresses, the constraints on our AI industry from AI algorithms and computational power, especially AI chips, have become clear,” noted AI expert and Baidu executive Wang Haifeng said.
Starting from Scratch
What would it require for China to patch its foundation deficit? Naturally, reinventing the wheel—or in this case reinventing TensorFlow—will not do. Rather, Chinese researchers will need to push the bounds of basic AI research, contributing new ideas to the global research community and building their own foundational platforms. The problem is that China, despite its strides in commercializing AI, does not appear to be making much progress in basic research. According to data compiled by Elsevier in partnership with CAS, the “citation impact” of Chinese AI papers remains significantly behind the numbers from Europe and the United States. Anecdotally, deep learning researchers point to one significant contribution from a Chinese lab in recent years—a paper out of Microsoft Research Asia’s Beijing lab in 2015 introducing “residual networks,” a training technique now widely used by other researchers—but most people struggle to name a second.
The most obvious reason for China’s struggles with basic AI research is old news: brain drain. Although efforts to train more Chinese AI researchers have succeeded, a huge fraction of those researchers—by one estimate, almost three-quarters—end up overseas. This stands in stark contrast to the United States, which is a massive net importer of AI talent. A major reason for this imbalance is the high quality of research labs in the United States, in both academia and industry. U.S. companies like Google and Facebook and universities like MIT and Stanford regularly top the charts of labs producing the highest volume of papers accepted at top conferences. (Perhaps not coincidentally, the lead author of the residual networks paper mentioned above has since moved from Beijing to a Facebook lab in California.)
Building up cutting-edge research capacity is a chicken-and-egg problem: The best researchers want to work with other outstanding researchers, giving a natural advantage to established labs and making it hard to bootstrap a great lab from scratch. High salaries and other incentives are often insufficient to overcome this dynamic, as demonstrated by Baidu’s struggles to retain high-profile AI talent.
Commercial incentives in China’s AI industry also reinforce the country’s basic research deficiency. Chinese tech companies largely underinvest in R&D, pursuing commercial applications over basic research. While the hype of AI has triggered a surge in attention from investors, investments have been mainly focused on applications that can be quickly commercialized. According to a survey conducted by EO Intelligence, a Chinese market research firm, AI ventures working in financial technology, service industries, and surveillance have received a disproportionate amount of investment. At the same time, ventures working on foundational components have been largely ignored by investors.
These incentives, according to voices in the Chinese AI community, have perversely influenced what AI researchers in China work on. “There are a lot of feasible projects, but most do not generate excitement. Experts pick the hottest project—this reflects a type of low self-esteem,” said Lu Ruqian, a CAS scholar and early pioneer in AI. "I believe this blind herd mentality is creating a dangerous situation," said Han Liqun, a professor at Beijing Technology and Business University.
Indigenous Innovation 2.0?
AI is not the first area of technology where Chinese experts have identified a national deficiency. Amidst the widespread adoption of information technology in the 1990s, government scholars bemoaned China’s reliance on foundational western IT systems and called for a concerted effort to build homegrown alternatives and wean China off western technology. This effort to harness “indigenous innovation” was placed front and center in the 2006–2020 National Medium- and Long-Term Plan for Science and Technology Development. Decades later, the results have been lackluster: China’s tech sector still has no credible competitor to Windows operating systems or Intel CPUs. The question is, will China’s push to patch its foundation deficit in AI be different?
One major difference lies in the commercial sector. The ZTE incident appears to have aligned industry with the government on the importance of building foundational technology. The addition of Huawei to the entity list this past spring might have been a second catalyzing event, once again reinforcing this point.
“If we do not master the core technologies, we will be building roofs on other people’s walls and planting vegetables in other people's yards,” said Alibaba founder Jack Ma shortly after Huawei was targeted. In September 2019 Alibaba’s chip subsidiary Pingtouge released its first dedicated AI processor for cloud computing. A month earlier, Huawei announced its first AI training chip and first open-source deep learning platform, MindSpore.
These developments suggest that the critical variable in whether China can catch up might not lie within China but in the United States. If the United States closes off its AI ecosystem, Chinese AI researchers have a meaningful incentive to develop their own platforms, no matter the costs.