Translation: Big Data Security White Paper 2018
China Academy of Information and Communications Technology, Security Research Bureau, July 2018
Blog Post
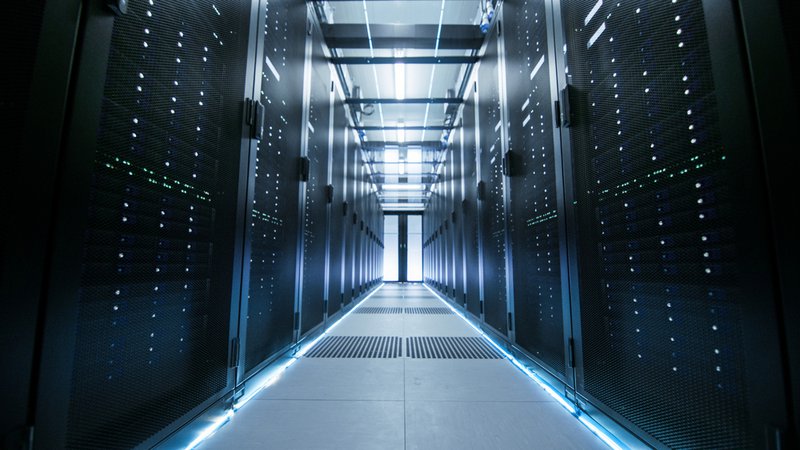
Shutterstock/ Gorodenkoff
July 31, 2018
Foreword
The global big data industry is presently in a period of rapid development. Technological evolution and innovation in applications are advancing with increasing speed. New forms of data storage, computing, and analysis technologies such as non-relational databases, distributed and parallel computing, machine learning, and deep mining have found a habitat for rapid evolution. At the same time that big data mining analysis in fields such as telecommunications, Internet, finance, transportation, and medicine is producing value in commerce and applications, it is also beginning to permeate traditional primary and secondary industries. Big data is progressively becoming a national basic strategic resource and an essential factor of production in society.
At the same time, big data security issues are increasingly apparent. Because big data entails centralized storage and management of very high-value data, it has become a major target of cyber attacks. Problems of big data ransomware attacks and data leaks are becoming more serious every day, and global big data security incidents occur frequently. In response to big data security demands, research and development (R&D) and production has emerged in security technologies and solutions in the form of programs and goods, but these lag in comparison to industry developments.
In the Politburo during the second national big data strategy team study session (国家大数据战略第二次集体学习), Chairman Xi Jinping said that China must: build a digital economy with data as a key factor; advance the integrated development of the real economy and the digital economy; and advance integration of the Internet, big data, artificial intelligence, and the real economy. At the same time, we must realistically ensure national data security. This demands that we must persist in the national overall security view; establish the proper cybersecurity view; persist in "protecting development through security and using development to support security"; and give full play to big data's important role in areas such as advancing industrial transformation and upgrading, and in raising national governance modernization levels. At the same time, we must: profoundly recognize the importance and urgency of big data security; clearly identify big data security challenges; actively face complicated and severe security risks; persist in emphasizing both security and development; accelerate the construction of a big data security safeguard system; and ensure national big data development strategy is successfully implemented.
This report begins from the starting point of the transformations brought about by big data, deeply discussing how big data security differs from traditional security. It then focuses on technological fields, giving an overview of big data security technology, and discussing security threats and security safeguard technology developments in the three areas of platform security, data security, and personal privacy security. Finally, based on the current conditions in big data security technology development, it assesses the future direction and offers recommendations for big data security technology development in order to provide a foundation and reference for big data industry and security technology development.
Table of Contents
Foreword
1. Understanding and Thinking About Big Data Security
2. Overall View of Big Data Security Technology
2.1. Big Data Platform Security
2.2. Data Security
2.3. Privacy Protection
3. Technological Problems and Challenges for Big Data Security
3.1. Platform Security Problems and Challenges
3.2. Data Security Problems and Challenges
3.3. Personal Privacy Security Challenges
4. The Situation of Big Data Security Technology Development
4.1. Big Data Platform Security Technology [Omitted]
4.2. Data Security Technology [Omitted]
4.3. Personal Privacy Protection Technology
4.4. The Present Conditions of Big Data Security Technology Development
5. Recommendations for the Future Development of Big Data Security Technology
5.1. Structure an Integrated Big Data Security Defense System From the Heights of an Overall Security View
5.2. Start From the Aspect of Attack Defense to Strengthen Big Data Platform Security Protection
5.3. With Key Links and Technologies as Breakthrough Points, Improve the Data Security Technology System
5.4. Strengthen Privacy Protection Core Technology Industrialization Investment, Taking Into Account the Two Important Priorities of Data Use and Privacy Protection
5.5. Emphasize Big Data Security Review Technology R&D, and Structure a Third Party Security Review and Assessment System
1 Understanding and Thinking About Big Data Security
The scale, processing, applications, and other aspects of big data have presented distinctive features compared with traditional data. Big data is a high-volume, structurally diverse, and timely form of data. To process big data requires employing new technologies such as computing frameworks and intelligent algorithms. Big data applications emphasize applying new concepts to assisted decision-making, discovering new knowledge, and even more so optimizing online closed-loop business workflows. From a security perspective, what influence have these new distinctive features of big data produced? We believe that:
1.1 Big data has already had a profound impact on the operating mechanisms of the economy, the mode of social life, and national governance. We must understand and solve big data security issues from the "great security" (大安全) perspective.
In the big data development process, resources, technologies, and applications are mutually dependent and develop in an upward spiral. Whether formulating commercial tactics, social governance, or national strategy, big data's ability to support decision-making is more and more emphasized. But big data must also be seen as a double-edged sword. It may not be possible to predict or prepare for the influence or destructive power of big data analysis and forecasting results. For example, when the analytical results from a U.S. fitness application's user fitness data were published online, the result was to leak suspected U.S. military secrets; this was previously unimaginable. In the future, intelligent policy decisions on the basis of big data may have even more important uses in economic processes, the life of society, and national governance. Big data may have profound influence on the national "11 kinds of security" (11 种安全).
It is therefore necessary to to examine big data security issues from the "grand security" perspective. We must examine the scene from the heights of the overall security view, break down traditional modes of thinking in security protection for key technologies, and build a big data security assurance system that touches on economics, law, technology, and other perspectives.
1.2 Big data is gradually evolving into a new-generation fundamental support technology. Big data platforms' own security is becoming an important influence factor in the security of big data and the integrated real economy.
Big data at present is becoming a general-purpose data processing technology. In addition to advancing innovation in artificial intelligence, virtual reality, and other new information technology applications, the Internet and big data are accelerating the advancement of digitization, networkization, and intelligentization through deep integration with the real economy. Even so, behind the booming development in informatization and industrialization, security issues are naturally emerging. As the methods of cyber attack on big data platforms change, attack objectives have changed from simply stealing data and paralyzing systems to intervening in and controlling analytical results. Attack effects have shifted from directly observable system downtime and information leakage to small and hard to detect analytical result errors, with results that could rise from a cybersecurity incident to industrial manufacturing accidents. Traditional cybersecurity technology based on monitoring, early warning, and response now faces trouble coping with these attacks. We must innovate in theory, counter constantly evolving forms of cyber attack, and design and construct a better big data platform protection system in order to raise the level of cross-sectoral foundational security assurance provision.
1.3 In the big data era, data value is maximized in the flow process. It is necessary to build a data-centric security defense system that suits the trend of cross-boundary (跨界) data flows.
In the big data era, data is a special kind of asset that, in the process of circulation and use, continually creates new value. In big data applications, therefore, data in motion is the norm and data at rest is the exception. At the same time, it can be foreseen that the future big data business environment will be more open, the business ecosystem more complicated, the roles in processing data more multifaceted, and the boundaries between systems, businesses, and organizations more blurry, leading to even richer and more diversified production, flows, processing, etc., for data. Data's frequent cross-boundary flows not only may lead to risks of traditional data leaks; they may also produce new risks. Especially in the data sharing channels, traditional data access control technology cannot solve cross-organization data permissions management and data routing issues. Relying only on written contracts or agreements, it is difficult to achieve monitoring and auditing of processing on the data recipient's side, which could easily lead to the risk of data abuse. The most prominent case is the Cambridge Analytica incident exposed this year. In the future, data sharing and flows will become a hard business requirement. Traditional static isolation security protection methods are thoroughly unable to fulfill data flow security protection needs. We must analyze and judge security risks from the angle of changing trends and build a data-centric, continuous data security protection system.
1.4 Big data promotes the vigorous development of new business models in the digital economy, but the masses face difficult tensions between increasing convenience from ubiquitous information services and protecting personal information rights.
In recent years in China, new business models in e-commerce, mobile payments, the sharing economy, etc., have developed rapidly. Information services built on the Internet, the mobile Internet, and the Internet of Things already permeate every aspect of social life and provide the masses with convenient, efficient, and constant services. For example, with inclusive finance, financial technology companies can use big data mining and analysis of personal data to better understand user needs and provide personalized services. Using big data to control financial risks can realize pipeline operations, minimize operating costs, improve service efficiency, and improve user experience. For example, an Internet financial services enterprise has coined the "310" personal credit services model, meaning "three minutes filling out a form, one minute evaluating a loan, and zero human intervention." Traditional credit services cannot compare with this user experience, and business costs have fallen from 2,000 RMB to 2.3 RMB per loan. Still, users enjoy this convenient service at the cost of selling (出让) their personal information rights. Information services such as everyday recommendations, personalized newspapers, and no-deposit car rentals are all based on big data mining and analyzing users' personal data, forming a user profile, and providing a customized service. With big data use as backdrop, however, ubiquitous data collection technology and specialized and diversified data processing technology make it difficult for users to control the conditions of collection and use of their personal information, and users' right to self determination over their personal information has naturally been weakened. Especially with regard to the increasing frequency of data sharing between businesses, processing data from different sources with big data's extremely strong analytical capabilities may resurface data that had previously undergone anonymization, resulting in the failure of today's de-sensitization technologies and direct threats to users' privacy security.
In sum, big data security is a comprehensive issue touching on areas such as technology, law, regulation, and social governance, and it can influence national security, industrial security, and people's legitimate rights and interests. At the same time, innovation in areas such as big data's scope, processing methods, and theories of application will not only bring about change in the security requirements of big data platforms, but also will drive changes in data security protection concepts and bring about requirements and expectations for high-level privacy protection technology.
2 Overall View of Big Data Security Technology
As mentioned above, big data security is a cross-disciplinary, comprehensive issue that can be researched from perspectives including law, economics, and technology. This report uses technology as an entry point to comb through big data's current security requirements and related technologies. It puts forward an overview of big data security; see Figure 1. In the process of plotting a big data security overview, we referred to domestic and international (e.g. NIST) big data technology reference frameworks and research. Considering big data platforms as the upper application layer providing storage and computational resources, they form the arena for tools in data processes such as collection, storage, computing, analysis, and display. We therefore start off from big data platforms to assemble the big data security overview.
In the overview, big data security technology systems are divided into the three layers of big data platform security, data security, and personal privacy protection, with each resting on the one before it. Big data platforms not only must ensure their own basic unit's security, they must also provide security assurance mechanisms for data and applications operating on the platform. Beyond platform security assurance, data security protection technology provides security protection strategies for data flows in enterprise applications. And privacy security protection is the protection of personal sensitive information on the foundation of data security.
Figure 1
Overview of Big Data Security Technology
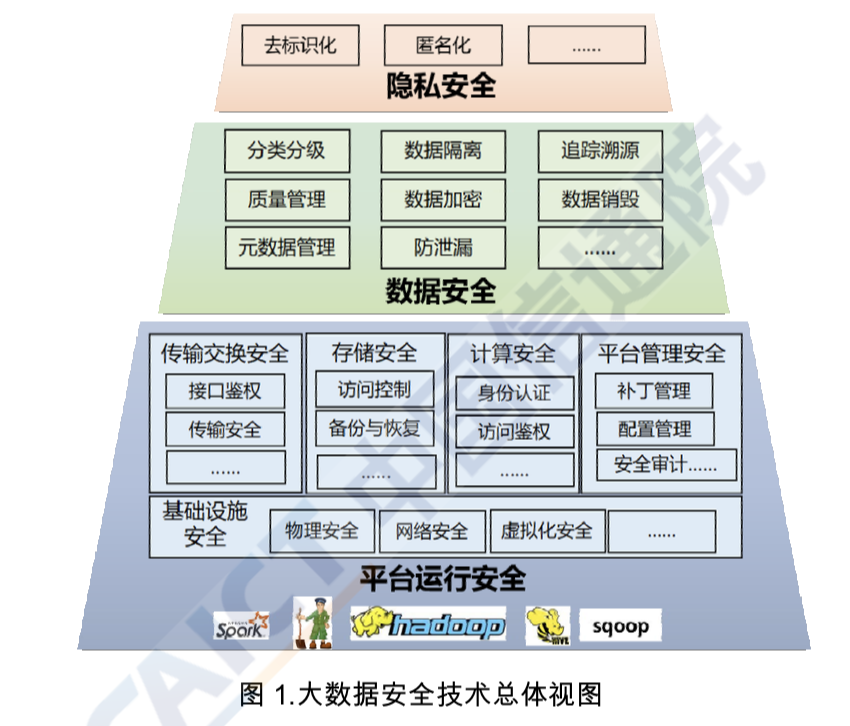
[Red block: Privacy Security—boxes: deidentification, anonymization, etc.
Green block: Data Security—boxes: classification, data segregation, source tracing, quality control, data confidentiality, data destruction, metadata management, breach prevention, etc.
Blue block: Platform operation security. Box 1: transmission and exchange security—interface authentication, transmission security, etc. Box 2: storage security—access control, backup and recovery, etc. Box 3: computational security—identity verification, access authentication, etc. Box 4: platform management security—patch management, configuration management, security audits, etc. Box 5 (bottom): infrastructure security—physical security, cybersecurity, virtualization security, etc.]
2.1 Big Data Platform Security
Big data platform security protects the security of resources and capabilities for transmission, storage, operations, etc. on a big data platform, including transmission and exchange security, storage security, computational security, platform management security, and infrastructure security.
Transmission and exchange security ensures security and controllability during the process of exchanging data with outside systems. It must utilize mechanisms such as interface authentication, verify the legitimacy of the outside system, and employ methods such as channel encryption to ensure confidentiality and integrity during transmission. Storage security entails backup and recovery of data on a platform, and data use access control mechanisms in order to prevent excess access. Computing modules provide relevant identity authentication and access control mechanisms to ensure that only legitimate users and applications can send data processing requests. Platform management security includes platform modules' secure deletion, resource security management, patch management, and security configurations. In addition, platform hardware and software infrastructure's physical security, cybersecurity, and virtualization security are among the foundations of big data platform security operations.
2.2 Data Security
Data security protection refers to platforms supporting data flow security capabilities, including data classification, metadata management, quality control, data encryption, data segregation, breach prevention, source tracing, data destruction, etc.
Big data spurs the data lifecycle to transform from the traditional single-link pattern to a complex multi-link pattern, increasing links for sharing, trading, etc. The arena and participant roles for data applications and are even more diversified. In the complex application environment, protecting sensitive data such as national important data, business secret data, and users' personal private data from leaks is the top requirement for data security. Large-scale, multi-source data is assembled in big data platforms, where a single data resource pool serves multiple data providers and data users at the same time. Strengthening data segregation and access control, and realizing data "usability without visibility," are new data security requirements in the big data environment. The results of using big data technology for large-scale data mining analysis may include sensitive information touching on national security, economic functions, social governance, etc., so security management when sharing and publishing those results must be strengthened.
2.3 Privacy Protection
In this report, "privacy protection" refers to de-identification, anonymization, ciphertext computation (密文计算), and other technologies to ensure that personal privacy or information a person does not wish the outside world to know is not breached during processing or transmission on the platform. Privacy protection refers to establishing a deeper level of security requirement for ensuring personal privacy rights on a foundation of data security protection. Moreover, we are also conscious that in the big data era, privacy protection is no longer merely the narrow protection of personal privacy rights, but also includes ensuring data subjects' personal information self-determination rights during the collection and use of personal information. In reality, personal information protection has already become a systematized project, for instance in product design, service operations, and security protection, and not purely a technological problem. But since this report emphasizes big data security technology, when it discusses protection of data subjects' personal rights and interests, we have chosen to simplify and begin research with privacy protection technology.
3 Technical Challenges Facing Big Data Security
Threats to big data security permeate all aspects of the big data supply chain, from data production to collection, processing, and sharing. Causes for risks are complicated and intertwined. There are external attacks and internal data leaks, vulnerabilities and management shortcomings, and new risks inherent in the use of new technology and new models. Moreover, traditional security risks have not been mitigated and continue to pose threats. This report will focus on and analyze three threats to big data security—big data platform security, data security, and personal data security—to determine a host of requirements for big data security.
3.1 Platform Security Problems and Challenges
3.1.1 Big data platforms using the Hadoop open source framework lack comprehensive security plans. Inherent mechanisms have limitations.
Currently, Hadoop has become the most widely used big data computing software platform, with its technological development integrated with the open source model. Hadoop was initially designed to manage large amounts of public web data, assuming that the cluster is always in a trusted environment and that it consists of trusted users using trusted computers. Therefore, the original Hadoop did not have a security mechanism in place, nor a security model or overall security plan. With increasingly widespread use of Hadoop, malicious behavior such as unauthorized job submissions, modification of JobTracker status, and data tampering continues to emerge. The Hadoop open source community has begun to consider security requirements and has added security mechanisms such as Kerberos authentication, ACL file access control, and network-layer encryption. These security features can address some of the security issues, but they still have limitations.
In terms of identity management and access control, when relying on Linux’s identity and rights management mechanisms, identity management only supports users and user groups, not supporting roles. It limits permissions to read, write, and execute, which cannot satisfy newer security requirements such as role-based identity management and fine-grained access control. In terms of security auditing, the Hadoop ecosystem only has distributed logs. There is no native security audit function, so external add-on tools are needed for log analysis.
In addition, the open source development model poses a potential security risk for the Hadoop system. When enterprises undertake R&D to develop new tools, they pay more attention to the realization of functions and performance increases than to the quality of their code and data security. As a result, open source components lack rigorous vetting procedures and security certifications, and are thus inadequately protected from component vulnerabilities and malicious backdoors. According to the Common Vulnerabilities and Exposures (CVE) vulnerability list, from 2013 to 2017, Hadoop revealed a total of 18 vulnerabilities, five of which were on information disclosure. Moreover, the number of vulnerabilities increases every year. The number of specific vulnerabilities over these last five years is shown in Figure 2.
Figure 2
Aggregation of Vulnerabilities in Hadoop, 2013-2017
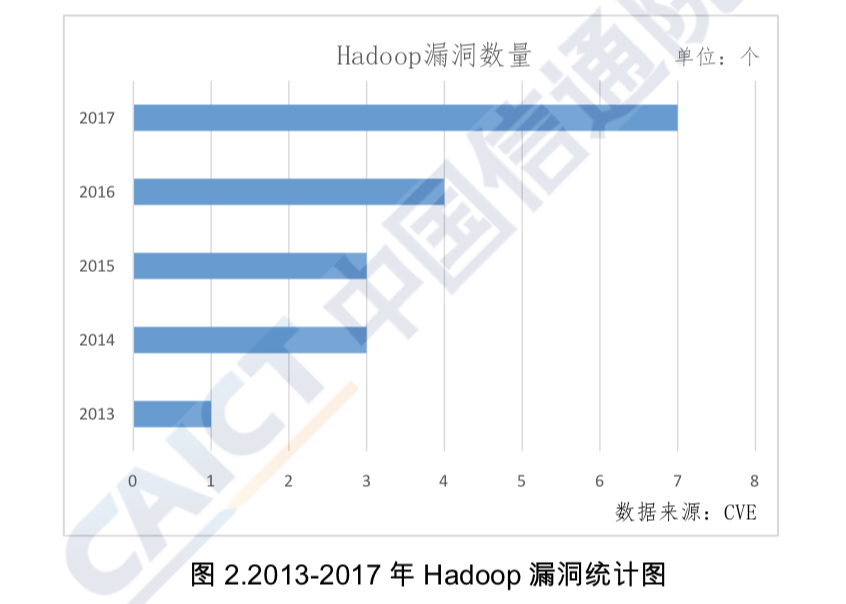
3.1.2 Big data platforms have many users and involve a diverse range of scenarios. Traditional security mechanisms may not meet platforms’ needs.
In situations requiring big data, data is aggregated from a more diverse range of channels, data types, user roles, and application requirements. Access control faces many new problems. First, the massive convergence of multi-source data increases the difficulty of access control policy formulation and authorization management. Both excessive authorization and insufficient authorization create serious issues. Second, the diverse nature of data, and the refining of user roles and requirements makes it more difficult to pinpoint how to describe objects. Traditional access control schemes often use data attributes (such as ID numbers) to describe the objects used in an access control strategy. Unstructured and semi-structured data cannot be refined in the same way, making it impossible to accurately specify the range of data that the user can access. This makes it difficult to determine the minimum authorization level. The complexity inherent in big data storage and flow makes it extremely difficult to implement data encryption. With such vast data stores, key management is also an urgent problem that needs to be solved.
3.1.3 Big data platforms’ large-scale distributed storage and computing model has made it difficult to configure increased security.
In the open source Hadoop ecosystem, functions such as authentication, rights management, encryption, and auditing are performed via configuration of related components, without configuration checking and evaluation tools. At the same time, large-scale distributed storage and computing architecture also increases the difficulty of security configuration. There are comparatively high technical requirements for security operations personnel. If an error occurs, it will adversely affect the entire system’s operations. According to analysis of Shodan’s search engine for Internet-connected devices, the improper configuration of big data platform servers has led worldwide to 5,120 terabytes of data leakage or potential data leakage risks. The countries with the most leaked data are the United States and China. The ransomware attack on the Hadoop platform at the beginning of this year did not take place by exploiting conventional vulnerabilities, instead relying on the platform’s insecure configuration to effortlessly manipulate the data.
3.1.4 Focusing on newer methods of launching cyberattacks on big data platforms, and how traditional monitoring techniques underexpose risk
The proliferation of big data storage, computing, and analysis has spawned many new and advanced forms of cyberattacks that have rendered traditional detection and defense technologies both grossly inadequate and unable to effectively resist intrusions. Traditional detection is based on real-time detection and matching of threat features based on a single point in time. However, looking in particular at advanced persistent threat (APT) attacks on big data, APTs take the long view, relying on long-term covert attack implementations, and do not have obvious features that can be detected by real-time analysis, making discovery much more difficult.
In addition, the fact that big data has low value density makes it difficult for security analysis tools to pinpoint value points. Hackers can hide their attacks within big data, making it difficult for traditional detection methods. As a result, APT attacks against big data platforms have occurred, and large-scale distributed denial of service (DDoS) attacks on big data platforms are not uncommon. Verizon’s 2018 Database Breach Investigations Report shows that 48% of data breaches are related to hacker attacks. Among them, DDoS, phishing attacks, and abuse of privileges are the main hacking methods. Specific data can be found in Figure 3.
Figure 3
Main attack methods resulting in data leakage.
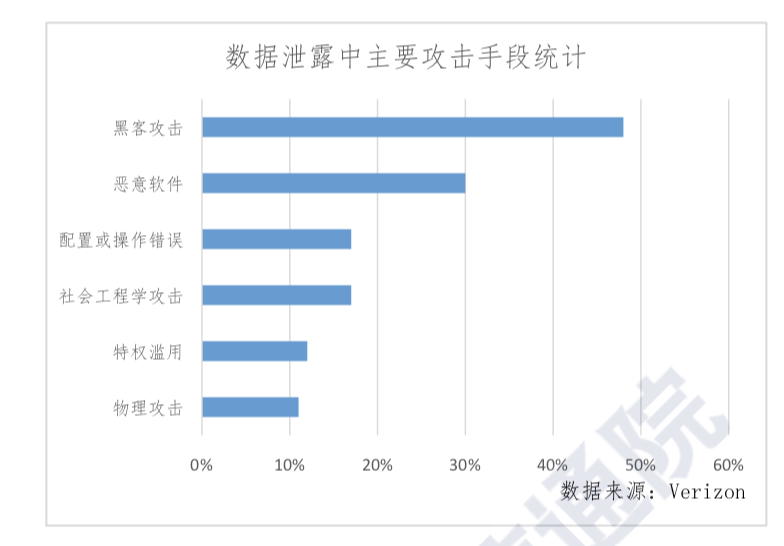
[Categories, from top: hacker attack, malware, deployment or operating error, social engineering attack, abuse of privileges, physical attack]
3.2 Data Security Problems and Challenges
In addition to the increasingly prevalent threat of data leakage, big data’s large volume and variety means threats to data security are new and different from threats to traditional data security.
3.2.1 The number of data breaches continues to grow, causing increasingly serious harm.
Big data has become a key target of cyber attacks due to its huge value and centralized data storage management. Ransomware attacks and data breach issues for big data are becoming increasingly serious, and major data security incidents frequently occur. Gemalto’s 2017 Data Breach Level Index report pegs the total number of data breaches worldwide in the first half of 2017 at 1.9 billion, already exceeding the total for 2016 (1.4 billion). This is an increase of over 160% from the second half of 2016. Figure 4 shows that the number of data breaches worldwide increased from 2013 to 2017. In 2017 alone, there were many major data breaches worldwide. Large-scale user data breaches that affected hundreds of millions of people included a U.S. Republican voter data firm [Deep Root Analytics] and the [Equifax] credit reporting agency. There have also been data breaches in China. In March 2017, probationary employees at JD.com colluded with hackers to steal 5 billion pieces of personal information related to transportation, logistics, and medical treatment, then sold them on the online black market.
In addition, the potential hidden dangers of data leakage are sobering. According to Shodan, as of February 3, 2017, there were 15,046 MongoDB databases in China exposed on the public web, which poses a major security concern.
Figure 4
Number of data breaches from 2013-2017
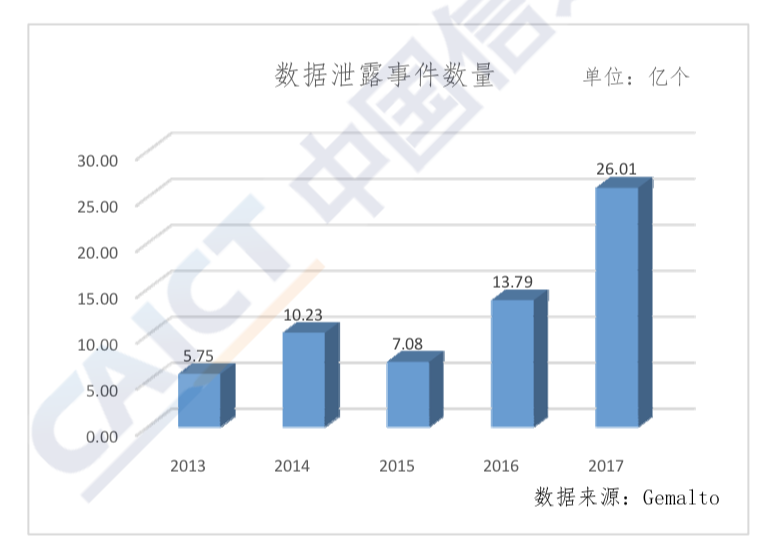
[Units: 100,000,000]
Source: Gemalto
3.2.2 Data collection is a new risk affecting decision analysis.
Over the course of the data collection process, big data’s large volume, variety, and diversity make it difficult to verify the data’s authenticity (真实性) and integrity (完整性). At present, there are no strict monitoring measures to ensure data authenticity and credibility. There is no way to identify and eliminate false or even malicious data. If a hacker relies on a cyberattack to inject dirty data into the data collection process, it will destroy the authenticity of the data. This will deliberately skew conclusions drawn from data analysis, and thereby allow hackers to successfully manipulate analysis results, as intended.
3.2.3. The issue of protecting confidentiality during data processing is gradually becoming more prevalent.
With the advent of the digital economy era, more and more enterprises or organizations need to be a part of the supply chain and carry out production activities dependent on data flows and cooperation. When enterprises or organizations are engaging in data collaboration and sharing, data will exit the organization and system borders, resulting in cross-system access or multi-party data aggregation to carry out joint operations. During the cooperation process, ensuring the confidentiality of personal information, trade secrets or unique data resources is a prerequisite for enterprises or organizations to participate in data sharing cooperation, and is also a problem that must be solved to ensure smooth-flowing data.
3.2.4 The complex nature of data flow paths makes traceability unusually difficult.
Big data application systems are complex. Frequent data sharing and exchanges interconnects data flow paths in a complex manner. From its generation to its destruction, data no longer follows a one-way, single-path simple flow model, and is no longer limited to flows only within organizations. Rather, data now flows from one data controller to another. It is more difficult to trace the full data path across data controllers or security domains in a heterogeneous network environment. With data traceability, it is especially difficult to ascertain the credibility of data tags. The fact that data tags and data content are intertwined raises more prominent issues including security concerns.
In the Cambridge Analytica incident in March 2018, because Facebook did not effectively manage nor hold accountable third-party data use, 87 million users’ data was abused. It also led to a sharp fall in stock prices and a decline in the company’s credibility, among other serious consequences.
3.3 Personal Privacy Security Challenges
Personal privacy harms from big data use are not limited to breaches. Big data's methods and capabilities of collection, processing, and analyzing data have also severely challenged traditional personal privacy protection frameworks and technological capabilities.
3.3.1 Traditional privacy protection technologies face failure due to big data’s strong analytical capabilities.
In a big data environment, companies that conduct relational analysis and deep mining of multi-source and multi-type datasets can recover anonymized data, and, going a step further, can identify specific individuals or gain valuable personal information. In traditional privacy protection, data controllers choose data protection technologies and parameters to protect personal data in individual datasets. They especially use technological methods such as de-identification (去标识) and masking (掩码). These cannot handle the privacy breaches produced by multi-source data analysis and mining.
3.3.2 Traditional privacy protection technology faces difficulties in adapting to big data's non-relational databases.
In the big data technological environment, data changes dynamically , and semi-structured and non-structured data form the majority, with non-structured data accounting for more than 80 percent of total data, and non-relational databases (NoSQL) the storage technology norm for gathering, managing, and processing big data. Non-relational databases presently use relatively lax access control mechanisms and imperfect privacy protection tools. Existing privacy protection technologies, for example de-identification and anonymization technologies, are more often used in relational databases.
4. The Situation of Big Data Security Technology Development
Faced with the aforementioned challenges and threats related to big data security, industry has undertaken targeted practices and investigations into security protection technology. This report looks at three aspects of the development of big data security technology: platform security, data security, and privacy protection.
4.1. Data Platform Security Technology
As market demand for big data security increases, the Hadoop open source community has added security mechanisms like identify authentication, access control, and data encryption. The commercial Hadoop platform has also gradually developed security components such as centralized security management, fine-grained access control, and other types of security components, as well as regular security updates.
[Note: At time of publishing, this translation omits three subsections under 4.1 and the entirety of 4.2, which generally focus on specific technologies, as opposed to policy and concepts. If a contributor translates these sections, DigiChina will edit and incorporate them. Here, we preserve and translate only the headings. –Ed.]
4.1.1. The Hadoop open source community has added basic security mechanisms, but these capabilities cannot meet real-world needs.
[Omitted]
4.1.2. Commercial big data platform solutions already have relatively complete security mechanisms.
[Omitted]
4.1.3 Common commercial security components can provide an extra layer of security for established big data platforms.
[Omitted]
4.2 Data Security Technology
[Omitted]
4.2.1 Sensitive data identification technology, necessary for data security monitoring, is gradually being automated.
[Omitted]
4.2.2 Anti-breach technology development is relatively mature and moves toward intelligentization.
[Omitted]
4.2.3 Structured database security protection technology is basically mature, but non-structured database security protection urgently needs strengthening.
[Omitted]
4.2.4 Ciphertext computation technology is a research focus because of multi-source computing confidentiality needs.
[Omitted]
4.2.5 Digital watermarking and data lineage tracing technology development lags needs.
[Omitted]
4.3 Personal Privacy Protection Technology
In the big data environment, data security technology provides the foundation for protecting confidentiality, integrity, and availability. Privacy protection, based on this foundation, ensures personal private information is not leaked or exposed to the outside world. Currently, the most widely used method is data desensitization, and academia has proposed homomorphic encryption (同态加密), secure multi-party computation, and other cryptographic algorithms for privacy protection, though these are not yet widely used.
4.3.1 Data desensitization technology is mature and is currently the most widely used privacy protection technology.
Data desensitization refers to modifying certain sensitive information in data through desensitization rules in order to achieve privacy protection of personal data. It is the most widely used privacy protection technology. Current desensitization technology can generally be divided into three types. The first, low-level, type of encryption method is a standard encryption algorithm that completely removes functional attributes. This type of algorithm is costly and is suitable for situations requiring a high degree of confidentiality and where there is no need to maintain functional attributes. The second type is data distortion technology, mostly using random interference or adding chaos. This is an irreversible algorithm that can create "fake data that looks real." It is suitable for group information statistics and/or situations where functional attributes must be maintained. The third type is a reversible replacement algorithm, which is both reversible and maintains functional attributes. It can be implemented through position changing, table mapping, algorithm mapping, and other techniques. Table mapping methods are relatively easy to use and can solve the problem of retaining functional attributes, but as the dataset grows larger, the mapping table grows larger, and use becomes limited. The algorithmic mapping method need not use a mapping table, and data conversion can be achieved through a self-designed algorithm (自行设计的算法). These kinds of algorithms are based on basic cryptographic concepts of self-design, with the usual method being to add certain changes to a foundation of a public algorithm. This method is suitable in situations where maintaining functional attributes and/or reversibility is required. When the data-using system is choosing desensitization algorithms, the balance between usability and privacy protection is the key, along with considerations of system running costs and fulfilling the system's functional requirements while adhering to the minimization principle to maximize user privacy protection.
4.3.2 Anonymization algorithms will become an effective way of solving privacy protection problems.
Data anonymization algorithms can, according to the specific situation, conditionally produce partial data or partial attributes of the data, including differential privacy, k-anonymity, l-diversity, t-closeness, etc. Problems to be solved by anonymization algorithms include: the balance between privacy and usability, performance efficiency, measurement and evaluation standards, anonymization of dynamically republished data, anonymity under multidimensional constraints, etc. Anonymization algorithms have received widespread attention in big data security for their ability to protect users' sensitive data from leaks in the published data environment while also ensuring the authenticity of published data. Anonymization algorithms currently still face many challenges needing urgent solutions. Algorithms' maturity and diffusion is still limited. Anonymization-related algorithms are one of today's hottest topics for research in data security. They have achieved rich research results and have been put to some practical uses, and future anonymization algorithms will see more and more applications in privacy protection.
4.4 The Present Conditions of Big Data Security Technology Development
Technologies related to platform security, data security, and privacy protection are improving, allowing us to solve the security issues and challenges laid out in the third chapter of this report. However, in order to counter new methods of cyberattack, protect new data applications, and meet the demands for increased privacy protections, higher standards and capabilities will be required.
In terms of platform technology, centralized security configuration management and security mechanism deployment basically meets the current security requirements of platforms. However, vulnerability scanning and attack monitoring technologies for big data platforms are relatively weak. In order to strengthen centralized security management in the Hadoop ecosystem, commercial big data platforms have patched security deficiencies in big data platforms through commercial common security components such as admission control, multi-factor authentication, fine-grained access control, key management, data desensitization, central auditing, and other security mechanisms. However, Hadoop is undergoing a phase of fast development, the authentication method relies on Kerberos, and its authentication center might become a system bottleneck.
In terms of technology to defend platforms from cyber attacks, current big data platforms still use traditional cybersecurity measures to defend against attack. This is insufficient for the big data environment, where an expansive defensive perimeter is vulnerable to methods of attack that conceal intrusion. Also, the industry has paid too little attention to the possibility that the next method of attack might come from the big data platforms themselves. Once new vulnerabilities appear, the scope of the attack will be immense.
In terms of data security, data security monitoring and anti-breach technology are relatively mature, but data sharing security, unstructured database security protection, and data breach traceability technologies need improvement. Currently, there are technical solutions to data breaches: technology can automatically identify sensitive data to prevent leaks; the introduction of artificial intelligence and machine learning is allowing breach prevention to evolve in an intelligent direction; and the development of database protection technology is also providing strong guarantees against data breaches. Ciphertext computation technology and data leakage tracking technology have still not developed to the point where they can meet real application demands, and it’s still difficult to settle the confidentiality guarantee problem of data processing and issues associated with tracking the flow of data. Specifically, ciphertext computation technology is still in the theoretical stage, and the computational efficiency does not meet real application requirements; digital watermarking technology cannot meet the needs of large-scale and fast-updating big data applications; and data lineage tracing technology requires further application testing and has not reached the stage of maturity for industrial applications.
In terms of privacy protection, the development of technology obviously cannot meet urgent demands for privacy protection. The protection of personal information requires the establishment of a system of guarantees rooted in legal, technological, and economic methods. Currently, the widespread use of data desensitization technology poses a challenge to multi-source data aggregation and could lead to failure. Emerging technologies like anonymization algorithms have few practical application case studies so far, and there are other general problems [with the technology], such as low computational efficiency and costly overhead. In terms of computing, there is a need for continuous improvements in order to meet requirements to meet protect privacy in a big data environment. As mentioned above, the clash between big data applications and personal information protection is not only a technical issue. In the absence of technical obstacles, privacy protection still needs legislation, robust enforcement, and regulation on collecting personal information for big data applications. Build a personal information protection system that involves multiple components such as government oversight, corporate responsibility, social supervision, and netizens’ self-discipline.
5 Recommendations for the Future Development of Big Data Security Technology
Big data is becoming a new driving force for economic and social development, and is increasingly influencing and impacting economic operations, lifestyles, and national governance capabilities. Big data security has been elevated to be on par with national security. Based on the challenges and issues big data security faces, and the development of big data security technologies, we propose the following suggestions for the development of big data security technologies:
5.1 Structure an Integrated Big Data Security Defense System From the Heights of an Overall Security View
Security is a prerequisite for development. It is necessary to comprehensively improve the security of big data security technologies, then build a comprehensive, three-dimensional defense system that runs through the cloud management end (云管端) of big data applications in order to meet the needs of both the nation’s big data strategy and its market applications. We first need to establish a security protection system covering the entire data life cycle, from collection to transmission, storage, processing, sharing and ultimately destruction. There needs to be comprehensive utilization of data source verification, encryption of large-scale data transmissions, encrypted storage in non-relational databases, privacy protection, data transaction security, preventing data leakages, traceability, data destruction, and other technologies. This needs to be combined with existing network information security technology to establish a well-ingrained defense system.
The second is to enhance the security defense capabilities of the big data platform itself. It should introduce identity authentication for users and components, fine-grained access control, security auditing for data manipulation, data desensitization, and other such privacy protection mechanisms. It is necessary to prevent unauthorized access to and data leakage from the system, while increasing the attention paid to inherent security risks involved in the configuration and operation of big data platform components. It is necessary to strengthen the ability to respond to emergency security incidents that occur on the platform.
The third is to transition from passive defense to active detection, using big data analysis, artificial intelligence, and other technologies to automate threat identification, block risks, and trace attacks. Ultimately, the goal is to enhance big data security from the bottom-up and advance capabilities to defend against yet unknown threats.
5.2 Start From the Aspect of Attack Defense to Strengthen Big Data Platform Security Protection
Platform security is the cornerstone of big data system security. From earlier analysis, we can see that the nature of cyberattacks against big data platforms is changing. Enterprises are facing increasingly serious security threats and challenges. Traditional defense monitoring methods will find it difficult to keep up with such changes in the threat landscape. In the future, research on big data platform security technology should not only solve the operational security problem, but also design innovative big data platform security protection systems that hone in on the ever-changing nature of cyberattacks. In terms of security protection technology, both open source and commercial big data platforms are in a stage of high-speed development. However, shortcomings still exist across platform security mechanisms. At the same time, the development of new technologies and new applications will reveal yet to be known platform security risks. These unknown risks require all industry parties to invest more in the security of big data platforms, starting from both offensive and defensive aspects, while paying close attention to how trends are developing for both big data cyberattacks and defense mechanisms. It is necessary to establish defense systems that are adapted to and build more secure and reliable big data platforms.
5.3 With Key Links and Technologies as Breakthrough Points, Improve the Data Security Technology System
In the big data environment, data plays the role of adding value, its application environment is increasingly complex, and all aspects of the data life cycle are facing new security requirements. Data collection and traceability have become prominent security risks, and cross-organization data cooperation is extensive and results in confidentiality protection requirements that trigger multi-source aggregation calculations. At present, technologies such as sensitive data identification, data leakage prevention, and database security protection are relatively mature, while confidentiality protection in multi-source computing, unstructured database security protection, data security early warning, and emergency response and traceability of data leakage events, remain still relatively weak. It is necessary to actively promote the integration of production, academia, research, and application, and accelerate the research and application of key technologies such as ciphertext computation in the improvement of computing efficiency. Enterprises should: strengthen the support of data collection, computing, traceability, and other key links; strengthen data security monitoring, early warning, control, and emergency response capabilities; take data security key links and key technology research as breakthrough points; and improve the big data security technology system; in order to promote the healthy development of the entire big data industry.
5.4 Strengthen Privacy Protection Core Technology Industrialization Investment, Taking Into Account the Two Important Priorities of Data Use and Privacy Protection
In the big data application environment, data use and privacy protection are naturally in conflict. The technologies such as homomorphic encryption, secure multi-party computation, and anonymization can achieve balance between the two and are ideal technologies to solving the challenge of protecting privacy during the process of big data applications. Advancements in privacy protection core technologies will inevitably greatly promote the development of big data applications. At present, the core problem of privacy protection technology is efficiency, with problems including high computing costs, high memory needs, and a lack of evaluation standards. Some research on the theoretical level is not yet widely applied in engineering practice. Dealing with privacy security threats such as multi-data-source or statistics-based attacks is difficult. In the big data environment, personal privacy protection has already become a widely-watched topic, and the increasingly widening privacy protection demands of the future will drive specialized privacy protection technology R&D and industrial application. To raise the level of privacy protection technologies in the big data environment, we must encourage enterprises and scientific institutions to study privacy protection algorithms such as homomorphic encryption and secure multi-party computation, and at the same time to advance application of data desensitization, auditing, etc., technological methods.
5.5 Emphasize Big Data Security Review Technology R&D, and Structure a Third Party Security Review and Assessment System
At present, the state has made a series of major decision-making arrangements for big data security. President Xi Jinping pointed out in the report to the 19th Party Congress that he wanted to promote the deep integration of big data and the real economy, and he has emphasized the effective protection of national data security. The 13th Five-Year National Informatization Plan proposed the implementation of the Big Data Security Project. It is foreseeable that the government regulation of big data security will be further strengthened in the future, the data security related legislation process will be further accelerated, big data security supervision measures and technical means will be further improved, and big data security supervision disciplinary efforts will be further strengthened. At the same time, building a big data security assessment system will become an effective measure to ensure the security of big data, through the formulation of big data security technology standards and assessment standards, establishing a big data platform and big data service security assessment system, and promoting third-party assessment agencies and personnel qualification certification, and other supporting management system construction, while from the perspective of platform protection, data protection, and privacy protection, effectively promoting the overall improvement of big data security assurance capabilities.