The Promise and Peril of Predictive Analytics in Higher Education
A Landscape Analysis
Policy Paper
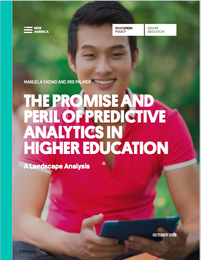
Oct. 24, 2016
Predictive analytics--using massive amounts of historical data to predict future events--is a practice that’s making it easier and faster for colleges to decide which students to enroll and how to get them to graduation. But using data in this way may make decision-making processes harder, not easier. That’s because predictive analytics can aid in discriminatory practices, make institutional practices less transparent, and make vulnerable individuals’ data privacy and security.
In a new paper, The Promise and Peril of Predictive Analytics in Higher Education: A Landscape Analysis, authors Manuela Ekowo and Iris Palmer describe how predictive analytics are used in higher education to identify students who need extra support, steer students in courses they will do well in, and provide digital tools that can customize the learning process for individual students. The paper also outlines the ethical concerns involved in using data to make predictions and its impact on underrepresented students.
“There are a number of examples of colleges using predictive data to make inroads in student success or operational functions. But that doesn’t mean we can or should turn a blind eye to the possibility that using this technology can go badly,” says Manuela Ekowo, policy analyst with New America’s Education Policy Program.
Ekowo and Palmer feature examples from several institutions, highlighting their predictive tools and successes to date. This includes an in-depth look at Georgia State University, an institution that has been recognized for its use of predictive analytics to help eliminate the achievement gap for its first-generation, Pell-eligible, black, and Latino students. Other institutions highlighted in the paper and of their use of predictive tools include:
- Temple University’s homegrown early-alert tool that analyzes the institutions’ data to identify students on the verge of dropping out of school, allowing Temple to provide robust advising to ensure students remain in college.
- Austin Peay State University’s course recommender system that matches students with courses and majors they are most likely to do well in and predicts what grade students will receive.
Despite these and many other colleges’ successes, using student and institutional data to make predictions about what may happen in the future is no easy feat. Profiling students could mean inhibiting or discouraging capable students from developing their talents or pursuing their interests. Transparency is also an issue when using predictive analytics. It is often not clear to those on campus why big data tools are used, how they were built (typically with private companies), or their limitations.
The paper also addresses the increasing challenges with keeping data private. Often data about students are shared within the institution and with outside vendors to create predictive models for early-alert and recommender systems. This sharing of data allows students and faculty to remain anonymous but this may be harder to do with the large data sets that predictive modeling often requires.
“For colleges that are just learning to use predictive analytics to make decisions, guarding against potential harms can be a struggle. But the stakes are too high to postpone asking these hard questions,” says Ekowo.
More information about these findings and others are in the full paper here.