Intervening with Care
Why Inclusive Education Policies Matter
Blog Post
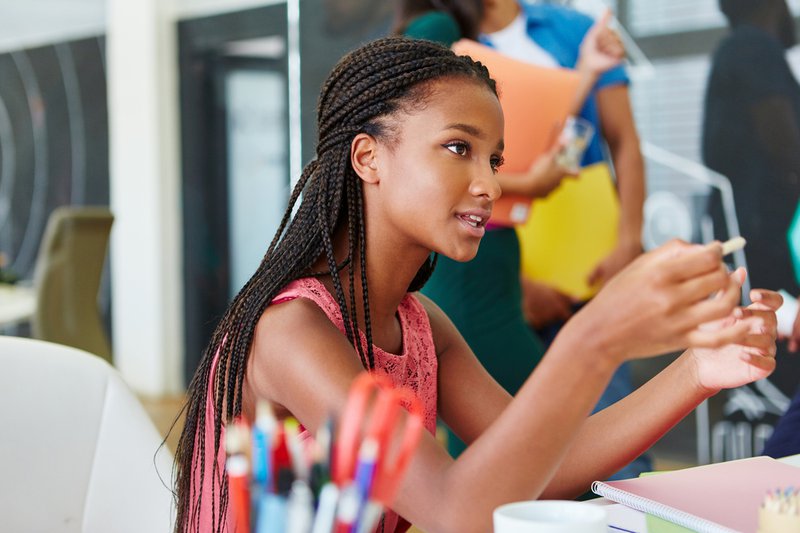
Shutterstock.com
May 17, 2018
As policy makers and researchers look to optimize outcomes for innovative social and educational policies, they must be mindful that their policies equitably meet the needs of affected communities. To that end, thoughtful policy leadership starts and ends with the following questions:
How do you intervene with care? And how do you design policies with different communities in mind to minimize unintended consequences that could actually lead to inequitable outcomes?
At April’s Millennial Public Policy Symposium, Millennial Public Policy Fellow Jenny Muñiz tackled these questions as they relate to education policy alongside two of her colleagues from the education program at New America— Abigail Swisher, whose work centers on college and career readiness through youth apprenticeship, and Ernest Ezeugo, whose research explores the use of predictive analytics and algorithms in higher education. Specifically, they presented a powerful argument as to why inclusive education policies matter and, building off this argument, offer strategies and solutions for addressing the needs and aspirations of low-income communities of color.
Youth apprenticeship, Swisher explained, is a partnership between a high school, a post-secondary partner, and an industry player to provide students with paid, on-the-job mentorship in combination with early access to post-secondary, for-credit classroom training. Apprenticeship programs, if done right, should provide individuals with a family-sustaining wage and a clear path forward in a specific industry. But as Swisher explicated when describing the equity dimensions of youth apprenticeship, there is a pernicious history surrounding vocational programs in the United States. This history is rife with discrimination, as Jeannie Oakes writes in her book Keeping Track, “an underlying function of vocational education has been to segregate poor and minority students into occupational training programs in order to preserve the academic curriculum for middle- and upper-class students.”
Algorithms have historically tended to benefit richer and whiter communities, while being forced onto lower income communities and communities of color.
Given this history, proposals for expanding apprenticeship opportunities that lead towards vocational occupations are rightfully met with increased scrutiny, even if the overarching intention behind such programs is good, and even considering that these programs show great promise. Muñiz summarized these quandaries by describing the thin line that can cause even well-intentioned policies to reinforce deficit perspectives and stereotypes that track low-income and minority communities. Similarly, Ezeugo warned of the potential unintended consequences of using algorithms and predictive analytics to forecast expected behaviors, a system that often disproportionately targets and tracks underserved populations.
Building on Virginia Eubanks and Cathy O’Neil’s research on the destructive power of algorithms, Ezeugo made the case that algorithms have historically tended to benefit richer and whiter communities, while being forced onto lower income communities and communities of color. Specific examples illustrate how algorithms can propagate racist stereotypes around black criminality, exclude job applicants based on gender, race, age, disability, or military service, and, ultimately, perpetuate poverty.
Despite these legitimate warnings, there is still incredible promise if strategies are approached thoughtfully and data is used both ethically and effectively. Critically, human interventions are needed to understand how data is unfairly deployed and interpreted, such that inequity-reinforcing biases are mitigated before being scaled across entire systems.
Swisher offered an equitable framework to achieve this goal. She emphasized, first, being able to articulate who programs are designed for, then making sure those individuals’ voices are at the table while creating programs. Throughout a program’s development, policy makers must take care to fully understand the scope of data being collected, quickly report the impacts of using this data, and adjust approaches before programs are scaled.
Innovations in education policy, founded on the ethical and effective use of data, can be used to teach us more about student success than we have ever known.
Ezeugo gave a specific example of a program at Georgia State University that has successfully implemented such an equity framework. The university created a program that uses predictive analytics to provide key insights on early interventions that can support struggling students. Rather than relying on entrenched, black-box algorithms to make decisions, the program continues to emphasize human interaction and iteration based on in-person feedback from students directly impacted by the program. Part of this strategy led Georgia State to invest in a robust counseling system, reducing the counselor to student ratio from 700 counselors per student to 300 counselors per student.
A key theme from this conversation is that innovations in education policy, founded on the ethical and effective use of data, can be used to teach us more about student success than we have ever known. This introduces new possibilities for promising interventions that would support individuals throughout their education. Being mindful of the risks, policy makers should embrace equitable and targeted frameworks that better deliver education outcomes to historically underserved communities.
This blog is part of Caffeinated Commentary - a monthly series where the Millennial Fellows create interesting and engaging content around a theme. For May, the fellows are recapping some of the many important conversations from their April Millennial Public Policy Symposium: New Voices and Ideas on Care, Community, Technology, and Civic Engagement.