Promoting Greater Fairness, Accountability, and Transparency Around Algorithmic Decision-Making in Content Moderation
Blog Post
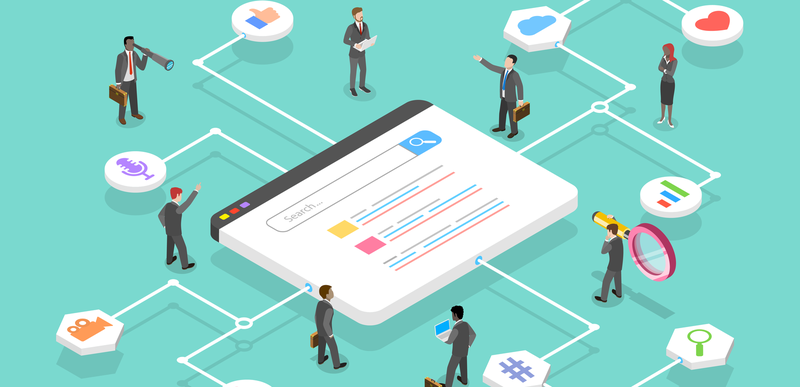
July 22, 2019
The rise and proliferation of digital platforms that enable users to create and share content has significantly altered how we communicate with one another and has democratized speech across the globe. However, positive or harmless content such as family photographs, humorous videos of cats, blog posts, and pieces of artwork is not the only type that proliferates on these platforms. With the rapid expansion of user-generated content, internet platforms have also had to deal with the spread of objectionable and even dangerous forms of content such as extremist propaganda, hate speech, and pornography.
In response, these platforms have introduced content policies and content moderation practices that aim to remove these objectionable, and sometimes illegal, forms of content from their products and services. Over the past few years, platforms have come under increased pressure from governments and the public to take a more proactive approach in order to identify and remove harmful content, and to do so quickly. As a result, companies both big and small have invested significantly in adopting or developing automated tools to play a greater role in their content moderation efforts.
However, despite significant investment, these tools have demonstrated a range of weaknesses and raised numerous concerns, including:
- Creator and dataset bias: The datasets that algorithmic models are trained on typically emphasize particular categories or definitions of speech, and also tend to emphasize speech that is English and Western-focused. The resulting algorithms can generally identify certain types of speech, such as hate speech directed at particular groups, but are not capable of being holistically applied to all sub-groups of global hate speech. In addition, the algorithmic models are trained on Western-focused language, despite the fact that millions of users reside outside the West and do not speak English. These inherent biases in training data can be exacerbated by algorithmic models. These biases threaten to further marginalize already disproportionately targeted communities around the world.
- Accuracy and reliability: Because the datasets that content moderation-related algorithmic models are trained on are not comprehensive and representative of the differences in human speech and behavior online across demographics, regions, and so on, these tools are limited in their accuracy and reliability when moderating global content. In addition, automated tools have been found to be most accurate when there are commonly agreed upon definitions and parameters around a category of objectionable content, such as those around child sexual abuse material. In most situations, however, obtaining such clear definitions and parameters is challenging, as human speech is highly subjective. As a result, erroneous content takedowns are common, posing threats to free expression online.
- Contextual understanding of human speech: Algorithmic decision-making tools are trained to make objective, rule-bound decisions. However, human speech is rich with nuances based on context and differing global and community norms. As a result, these tools are limited in their ability to detect and moderate content, and this often results in erroneous and overbroad takedowns of user speech, particularly for already marginalized and disproportionately targeted communities.
- Transparency and accountability: Currently, very few internet platforms provide meaningful transparency and insight around how automated tools are deployed for content moderation; how these tools are developed, refined, and tested; how accurate these tools are; and how much user expression these tools have correctly or erroneously removed. In some cases, this is because automated tools are black boxes, and thus comprehending their decision-making practices is a challenge. In other cases, however, this is because companies are not disclosing adequate information.
In its most recent report, New America’s Open Technology Institute (OTI) explores how small and large internet platforms have deployed automated tools for content moderation purposes, and how internet platforms, policymakers, and researchers can promote greater fairness, accountability, and transparency around these algorithmic decision-making practices. The report includes case studies that explore how three platforms—Facebook, Reddit, and Tumblr—have deployed automated tools to moderate content, and what challenges they have faced. The recommendations presented in the report include:
- Policymakers need to educate themselves on the limitations of automated content moderation tools, and should encourage companies to establish responsible safeguards and practices around deploying these tools, rather than placing pressure on platforms to rapidly remove content in a manner that generates negative consequences.
- Companies need to take a more proactive role in promoting fairness, accountability, and transparency around algorithmic decision-making related to content moderation. This is a vital process that can and should take many forms, including:
- Disclosing more information around the inputs, outputs, ethical uses, and accuracy rates of their algorithmic models
- Publishing information around the scope and volume of content takedowns by automated tools, as well as subsequent appeals of these content takedown efforts, in corporate transparency reports
- Providing notice to users who have had their content or accounts suspended or removed, and offering users robust appeals processes
- Providing researchers with access to algorithmic models for evaluation and assessment
- Investing more resources into developing algorithmic models that are diverse and that can account for variations in speech and online behavior across regions, communities, etc.
- Research on algorithmic decision-making in the content moderation space needs to be more robust and should seek to test and compare how effective automated content moderation tools are across a range of factors, including platforms, domains, and demographic attributes.
- Automated tools should supplement, not supplant, human roles. Keeping a human in the loop during the content moderation process will help safeguard freedom of expression, and foster and maintain fairness and accountability in the content moderation process.
This is the first in a series of four reports that will explore how internet platforms are using automated tools to shape the content we see and influence how this content is delivered to us.