What U.S. Policymakers Should Know about California’s and Colorado’s AI Legislation
Blog Post
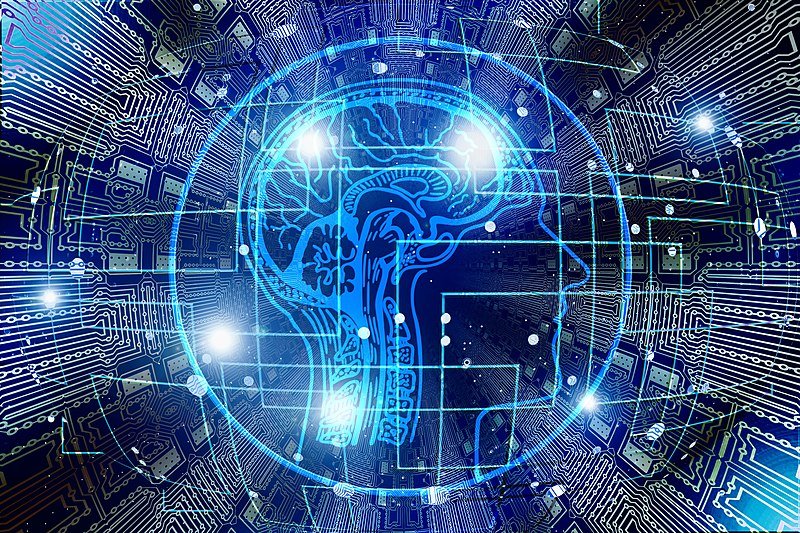
Aug. 26, 2024
In the rapidly evolving landscape of artificial intelligence (AI), state and federal U.S. legislators are grappling with how much the technology should be regulated as well as what such regulation should entail. Is there a form of AI governance that effectively balances innovation with safety? What aspects of the technology should be regulated? How can we ensure that policies at the state and federal levels help the American public reap the benefits of AI? These are all questions that policy leaders across the United States should be considering.
California and Colorado recently emerged as pioneers in AI regulation, each working on or passing landmark legislation aimed at governing AI systems. These states approach AI governance quite differently. Colorado's AI law, SB 24-205, takes a broader, consumer-centric approach that offers more immediate protections for individuals while potentially allowing for greater flexibility in AI development and deployment. California’s AI bill, SB 1047, however, hones in on "frontier models"—cutting-edge AI systems with the potential for both significant benefits and risks. It establishes a regulatory framework with particularly burdensome requirements that’s meant primarily for developers and deployers of these advanced AI models.
To craft and implement effective AI regulations, U.S. state and federal policymakers must carefully balance fostering innovation, ensuring accountability, and protecting individual rights. This balance should be achieved without imposing overly burdensome requirements that could stifle progress, particularly for open-source developers. The two pieces of legislation under consideration take different approaches to three crucial aspects of regulating digital technology for the public good: effects on the open-source ecosystem, civil rights protections, and algorithmic transparency and accountability.
1. Effects on the Open-Source Ecosystem
Out of the two pieces of legislation, California's bill is most honed in on impacting open-source AI, and its stringent regulations and requirements imposed on "frontier models" could severely hinder open-source AI development and innovation. The legislation creates a regulatory environment that could significantly discourage the collaborative, distributed development processes underpinning open-source projects.
Section 22603 of the bill requires developers of covered models to implement safety and security protocols, conduct impact assessments, and provide detailed documentation. Notably, developers must implement a protocol that "provides reasonable assurance" they won't produce models posing "unreasonable risk of causing or enabling a critical harm." This particular requirement is fundamentally flawed.
As Princeton computer scientist Arvind Narayanan argues, AI safety is "not a model property," but it depends on deployment context and broader socio-technical systems. The bill’s approach to safety could unreasonably burden developers, especially in the open-source community, by disincentivizing them from widely distributing their models. Developers could end up restricting access to their models or abandoning open distribution altogether for fear of being held responsible for the models’ potential misuse or unintended consequences in applications, which those developers didn’t anticipate or approve. This chilling effect on model sharing could significantly impact the collaborative nature of open-source AI development, where iteration and improvement often rely on broad access to existing models.
These disincentives for model sharing and distribution could lead to a more closed, proprietary AI development ecosystem, further constraining competition and limiting the benefits of open-source collaboration.
Meanwhile, Colorado's law, while not directly addressing open-source AI, provides exemptions for smaller deployers and certain research activities. This could indirectly benefit open-source projects by reducing regulatory burdens on smaller entities and academic researchers, potentially supporting a more open environment for model sharing and collaborative development.
These contrasting approaches to open-source AI highlight a crucial challenge in AI regulation: balancing safety and accountability with the need for open innovation. California's approach, while aiming to address potential risks, could inadvertently concentrate AI development into a couple institutions and companies that have the resources to navigate complex regulatory requirements. Colorado's more flexible approach might better preserve the diverse, collaborative ecosystem that has driven much of AI's—and the internet’s—rapid progress.
2. Civil Rights Protections
Both pieces of legislation strongly emphasize preventing algorithmic discrimination, but their approaches to this issue differ significantly.
California's bill requires developers to implement measures to mitigate risks of algorithmic discrimination, and it mandates disclosing such risks to deployers and the Frontier Model Division. However, the broad scope and stringency of these requirements may inadvertently limit certain AI developments aimed at improving representation and addressing bias. For instance, developers often fine-tune large language models to make their outputs more representative of diverse populations. This process involves additional training on carefully curated datasets to adjust the model's "voice" or to mitigate biases. Under the California bill's strict safety protocols and documentation requirements, such fine-tuning efforts could be classified as creating new "covered model derivatives," potentially subjecting them to burdensome regulations and discouraging this important work.
Colorado's law takes a different approach by explicitly defining "algorithmic discrimination" and requiring deployers to notify consumers when AI systems are used in consequential decisions. It also provides consumers with the right to appeal adverse decisions and correct incorrect data. This consumer-centric approach could give more immediate and tangible protections for individuals affected by AI-driven decisions.
The effectiveness of these different approaches in addressing civil rights concerns remains to be seen. California's comprehensive requirements could lead to more thorough considerations of potential discriminatory impacts during the development process. However, the high regulatory barriers could also discourage smaller organizations or startups from developing AI systems, limiting innovation and viewpoint diversity in this crucial area.
Colorado's focus on consumer rights and transparency may empower individuals to challenge discriminatory outcomes more effectively. By providing clear mechanisms for appeal and data correction, it could create a more dynamic system for addressing discrimination as it occurs. However, this reactive approach may not be as effective at preventing discriminatory outcomes in the first place.
The divergent strategies of these two states in addressing algorithmic discrimination highlight the complexities of regulating AI to protect civil rights. If these approaches are enforced as written, it will be crucial to monitor their impacts on both the development of AI systems and the real-world outcomes for individuals and communities affected by AI-driven decisions.
3. Algorithmic Transparency and Accountability
Both California and Colorado center transparency and accountability in their AI regulations, but they do so with different points of focus and potential outcomes. California’s approach centers on developer accountability, requiring detailed documentation of model training, limitations, and intended uses. The bill also mandates regular audits and certifications of compliance. While this method may increase transparency for large-scale models, it could also create barriers to entry for smaller developers and open-source projects for whom these extensive requirements are unrealistic or resource-prohibitive.
Colorado’s law emphasizes consumer-facing transparency, requiring clear disclosures when AI systems are used in decision-making processes. It also mandates that deployers maintain and periodically update public statements about their AI systems and risk management practices. This approach could empower consumers with more information about the AI systems affecting their lives, enabling more informed decision-making and scrutiny.
The California approach, with its focus on comprehensive documentation and auditing, could lead to more robust and thoroughly-vetted AI systems. But the cost and administrative burdens of compliance could stifle innovation, particularly among smaller organizations and open-source projects. This could result in a less diverse AI ecosystem with development concentrated among larger tech companies with the resources to meet these requirements.
Colorado's consumer-centric transparency measures could foster a more dynamic marketplace for AI applications where consumer awareness and choice drive improvements in AI systems. These requirements may also spur competition among companies in developing and showcasing best practices as well as in the quality and clarity of their transparency efforts. However, this approach may be less effective at catching potential issues before they impact consumers, as it relies more heavily on post-deployment scrutiny and feedback.
What These Pieces of Legislation Mean for AI Regulation in the United States
California’s and Colorado’s approaches to AI governance highlight the complexity of regulating AI at the state level. California's focus on advanced AI models risks hindering innovation, especially for open-source projects, due to costly compliance requirements, and it may inadvertently centralize progress into a handful of large players. Colorado's consumer-oriented policy offers broader protections and flexibility, potentially adapting better to rapid AI progress by regulating impacts rather than technical specifications.
These bills will likely serve as models for other states and potentially federal legislation. The stark differences between them underscore the need for a nuanced, multi-faceted approach to AI regulation that balances innovation, accountability, and individual rights without imposing overly burdensome requirements that could stifle progress, particularly to open-source developers.