The Future of AI Tutoring in Higher Ed
Brief
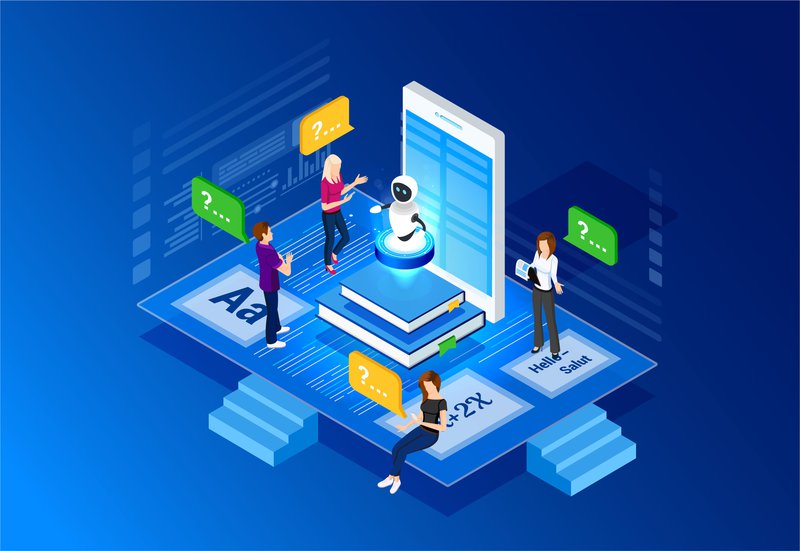
Marina Shevchenko/Shutterstock
April 4, 2023
Introduction
The increase in remote learning brought about by the COVID-19 pandemic created new demand for tutoring options. As rapid advances in artificial intelligence (AI) continue, AI-based tutoring systems promise a way to overcome the availability, cost, and location obstacles students may face with obtaining human tutoring. Once this technology reaches the point of cost-effective deployment, it could facilitate affordable, on-demand tutoring opportunities for millions of low-income students, students of color, and other underserved students, who may have previously been deterred from more traditional in-person or online tutoring programs opportunities by the price or by other obligations (e.g., jobs, caregiving) rendering them unavailable during these programs’ limited, set hours.
While computer scientists and other researchers have worked to apply artificial intelligence in developing “intelligent tutoring systems” over the past three decades, there has been relatively little impact on real-world student tutoring. Usage of currently-available AI tutoring tools in higher education is low, but institutions are tracking their potential for future use. However, the recent rapid improvement in the abilities and quality of generative AI technology—including the much-discussed ChatGPT, text-to-image models like Stable Diffusion, voice-to-text applications like Whisper, and others—suggests that AI tutoring systems may become advanced enough to be more widely used by universities sooner than expected. In some learning domains, such as medical training, there are already reports that AI tutoring is outperforming human tutoring. While it appears unlikely that AI will replace human tutors any time soon, in the near term, AI tutoring may be more frequently used as a complement to human tutors by providing personalized learning and practice modules, in which students engage with various AI personalities to create a realistic problem-solving experience.
The use of AI in tutoring, like other applications, comes with the challenge of protecting privacy. Emerging technologies often present new privacy risks, but privacy will be of particular concern with AI tutoring, considering both the large amounts of student data needed to train algorithms and the highly personal information that AI tutoring systems may collect during sessions, including detailed information about students’ feelings, behaviors, and activities.
Another major factor in developing AI tutoring systems is the necessity of avoiding bias. While AI can potentially identify and reduce biases, it can also perpetuate them at scale if the algorithms and the data used to train them are biased. Problems have already appeared with the use of AI for predictive analytics at colleges. For example, in 2020, the University of Texas at Austin ended the use of GRADE—a machine-learning system for evaluating computer science PhD applicants—due to concerns that the system, which automatically scored applications on their likelihood of approval by quantifying numerous factors (such as the names of colleges on their resume and specific words used in their letters of recommendation), could lead admissions committee members to unjustly overlook qualified applicants.
A Brief History of “Intelligent Tutoring Systems”
Beginning in the 1960s, initial use of computers for teaching and tutoring purposes in education was known as “Computer-Aided (or Assisted) Instruction” (CAI). Early programs such as PLATO (Programmed Logic for Automatic Teaching Operations) included the ability to parse free-text entered by the student and provide responses to certain incorrect answers.
CAI systems progressed to more complex and adaptive tutoring applications, eventually termed “Intelligent Tutoring Systems” (ITS). Early ITS technology had the capability of generating learning material, followed by a problem to be solved tailored to a specific student’s ability, and provided specific feedback based on the answer, using remedial techniques if the answer was incorrect. These systems were generally designed around three models: domain knowledge (an expert knowledge model), learner knowledge (a model of the student), and knowledge of teaching strategy (a tutoring model), along with a user interface.
SCHOLAR, developed in 1970, is often regarded as the first ITS. Constructed around a semantic network model (a store of information with relations between concepts), the program engaged students in a dialogue on geography, with the student and program communicating back-and-forth using natural language—the program posing a question to the student (or answering the student's question), then giving feedback based on the correctness of the student's response.
Other early ITS systems, such as SHERLOCK—commissioned by the Air Force for use in electronics troubleshooting training—used a different model: coached practice. In these models, tutoring is provided in the context of students engaging in problem-solving tasks, reflecting a “learning-by-doing” model of education.
The first ITS to be used in a number of real-world classrooms was the Cognitive Tutor (now known as MATHia). Developed over the span of more than a decade by cognitive psychologists at Carnegie Mellon University, MATHia is used in middle school and high school mathematics to provide individualized, real-time feedback and assessments.
While these earlier ITS programs were sometimes classified as using artificial intelligence, it is only in recent years that what we now conceive of as AI has been applied to computer tutoring systems. While AI is a very broad term covering many specializations and subsets, it is distinguished by the ability to modify its algorithms and create new algorithms in response to training data and user inputs. “Machine learning” is often characterized as AI (or used as a synonymous term), but machine learning is only one element of AI: algorithms that can dynamically modify themselves when exposed to more data. A subset of machine learning, “deep learning,” uses neural network processing of data—a computational system consisting of a set of interconnected nodes, modeled loosely on the human brain—and has been a major part of the recent advances in AI.
Some modern, purpose-built AI tutoring systems are now in use at colleges, including Walden University’s Julian, developed using Google Cloud's AI capabilities, and Georgia Tech’s Jill Watson. These AI tutors give students personalized assignments, feedback, and guidance. Beyond text-based AI tutors, work is being done on creating immersive tutoring environments populated by intelligent agents, including one developed by IBM Research and Rensselaer Polytechnic Institute to teach Mandarin.
Though AI has been rapidly developing in the past few years, implementation of AI tutoring has been limited. While the shift to fully online learning caused by the COVID-19 pandemic accelerated the use of chatbots by universities, expanding their use for limited tutoring purposes, these chatbots have mainly been used for informational and technical support, such as answering common university services questions or routing questions to the appropriate teaching assistant. However, the release of ChatGPT in November 2022 demonstrated a significant advancement in AI development, introducing a chatbot capable of generating informative responses and holding intelligent-sounding conversations. ChatGPT can already be used to provide a form of tutoring for students by explaining difficult concepts, though there are important questions about the correctness of ChatGPT’s answers, as the technology has been known to make up “facts.”
Privacy
The scale and scope of AI create a unique set of privacy challenges when applying this technology to tutoring. AI requires not only the processing of huge amounts of training data, but also the use of that data to learn and develop adaptive models to make predictions and decisions—often without transparent, easily explainable processes.
A key privacy concern for advanced AI tutoring and mentoring systems is the finding that people are likely to interact with anthropomorphized AI interfaces as if they were human. As such, students may develop trusting relationships with AI tutors, and share more personal information than they would through traditional technologies. Unlike human tutors, AI has the potential to easily collect, analyze, and store all of this highly personal information. Informed consent for data collection and use under these circumstances could be problematic when a sophisticated AI tutoring system has learned to understand the student and can potentially manipulate their preferences about data use.
While the use of AI presents privacy challenges, it should also be noted that in some ways, AI may help to preserve privacy. In situations where AI is implemented, fewer people will need to access and work with the underlying, unencrypted data. AI may also allow individuals to receive highly personalized services based on privacy preferences that AI systems learn over time.
As AI tutoring becomes more scalable, it is likely that third-party Educational Technology (edtech) companies will contract with higher education institutions to provide AI tutoring services. This may lead colleges to be less than transparent about the methods and choices of the AI tutoring products they use. By contracting with third-party providers, colleges may also lack ownership and access to the data AI tutoring products obtain from their students. While this data can be important in further improving the personalization and tutoring ability of AI applications, there must be clear data use and retention policies in place to ensure that data is stored only as long as necessary. With AI tutoring systems, there may be the desire to store and use long-term data following cohorts of students over many years, collecting information on their interactions with AI tutoring during K-12 through to higher education.
As the use of AI for tutoring and other applications grows in higher education, schools will need to ensure that policies and procedures are in place to address concerns about new AI uses of student data. This will include building expertise and capacity to deal with the privacy complexities of AI. Only a minority of universities have a Chief Privacy Officer in charge of creating and enforcing privacy policies, and few have a full-time staff person in a privacy role.
Bias
Theoretically, an AI tutor could respond in an unbiased way to all students, adapting to each student’s needs at a granular level, while potentially avoiding the possible pre-existing biases of human tutors. However, whether AI helps reduce bias or ends up crystallizing existing biases will depend greatly on the design of the AI tutor itself. There are numerous examples of bias in the design of algorithms and AI systems leading to harmful consequences, such as denying women job opportunities, overestimating black criminal recidivism, and inappropriately prioritizing health care based on race.
Algorithmic biases often result from the underlying data used to train AI algorithms. Bias is introduced by the way training data is collected or selected. Representational bias is one type of data bias that occurs when a group is under-sampled in the training data. For example, college graduation predictions have been found to be poor for indigenous students due to inadequate data.
Data later generated by users of the AI system can also introduce bias in a feedback loop-like manner. Further, adaptive algorithms used in AI change constantly—to the extent that those who create them often cannot fully explain the results they generate.
Designing an AI tutoring system often involves a more thorough analysis of learner needs than typical instruction, but AI models can potentially make incorrect assumptions about student learning. Detection of learning states is another aspect of ITS that may further advance under AI. A student’s emotional state is evaluated during a learning session, such as confusion or high interest, which the system uses in creating subsequent responses to the student. Identifying emotional states and intrinsic motivation accurately will likely be key to effective AI tutors, but developing these abilities will present bias challenges.
It is not solely training data and algorithmic design that lead to bias in AI. There is also the societal context in which AI is used: what institutions and people use the algorithms and for what purposes, and under what conditions and circumstances. Systemic biases from policies and practices of institutions can result in certain groups—namely, historically marginalized and underserved communities—being disadvantaged. Developing unbiased, trustworthy AI for mentoring and tutoring purposes in higher education will require taking into account all of these human and systemic factors—and identifying and accounting for them will require a broad range of stakeholders from various fields and communities.
The Tech and Regulatory Future of AI Tutoring
While current AI tutoring applications like Julian are primarily text-based, the interfaces and visualization capabilities of these systems will likely continue to advance. The future of AI tutoring may eventually see increased use of extended reality, or XR—an umbrella term that covers virtual reality (VR), augmented reality (AR), and mixed reality (MR). XR will present a number of additional privacy and equity challenges. Collection of biometric data, such as eye-tracking, enables companies to collect highly personal data about preferences and thoughts through unconscious responses to visual cues. Bias concerns will remain with the structuring of lessons and AI responses in XR, with additional issues relating to the design of avatars, environments, and other aspects of XR. Additionally, depending on their cost and availability, XR technologies could widen inequalities.
While there have been increasing calls for legislation, the US currently has no comprehensive law regulating AI. The Federal Trade Commission (FTC) holds companies using algorithms accountable under Section 5 of the FTC Act. In its 2016 report, Big Data: A Tool for Inclusion or Exclusion?, and in guidelines issued in 2020 and 2021, the FTC stresses that AI must be transparent and its use fair. Many states have also introduced or passed bills regulating artificial intelligence.
Additionally, the advance of AI has led to the development of a number of guidelines and principles documents, including the recently released NIST AI Risk Management Framework and a “blueprint” for an AI bill of rights recently released by the White House. Guidelines and certifications specific to AI educational technologies are being developed as well, such as the Edtech Equity Project’s Prioritizing Racial Equity in AI Design certification, which takes into account racial bias in edtech development and design.
In recent years, critics have urged the Department of Education to update the Family Educational Rights and Privacy Act (FERPA) to meet data privacy and security needs created by the advancing use of technology. The law could be amended to provide explicit consideration of the personal information obtained through AI technologies. This data could be far more personal than attendance records—including detailed information about students' thoughts, behaviors, and personal characteristics—and has the potential for more negative consequences for students if released. Students should be directly informed about what information AI tutors and chatbots store, and what is being done (or could be done) with the information. This may prove difficult, given the complexity of AI algorithms and their frequent lack of transparency and explainability. How FERPA applies to AI technologies—and how FERPA’s provision for providing students access to information about them that is that being stored and used will work in AI environments—are important questions in need of clarification.
Conclusion
AI has the potential to provide on-demand tutoring that would give students personalized assignments and guidance whenever and wherever they needed it. However, AI tutoring should be part of larger ongoing debates concerning AI privacy safeguards and how to overcome bias. AI tutoring systems can capture much more personal data, at greater granularity, than humans can. This data can then be used in ways that are often not transparent and easily explainable. While AI tutoring tools can potentially help close achievement gaps, their ultimate effect depends on proper development and implementation. AI tutoring applications should be designed inclusively, with consideration for diverse gender, race, class, ability and culture groups. Implementing trustworthy AI tutoring and mitigating bias will also require conducting ongoing assessments as real world use expands to identify situations in which these systems are generating harmful or inaccurate results.