What Do State Privacy Laws Mean for the Ad Tech Industry?
Brief
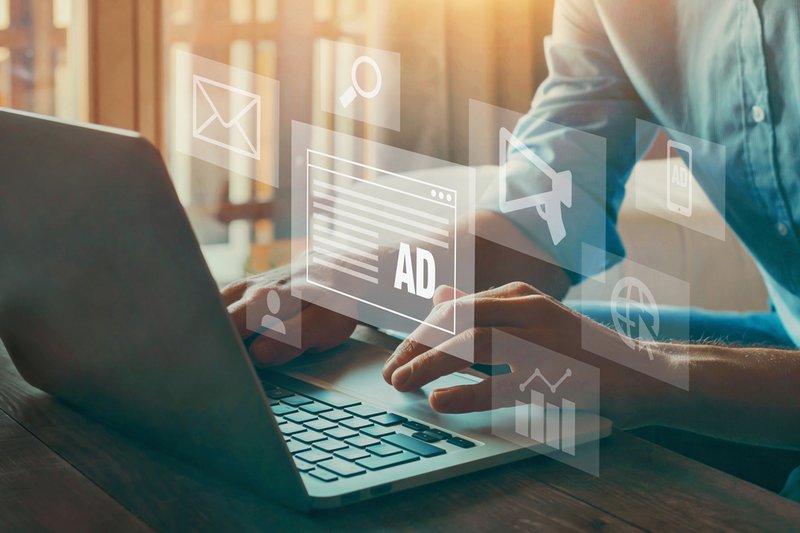
Shutterstock
Aug. 17, 2021
Introduction
In the absence of comprehensive federal privacy legislation, states have passed their own privacy laws to protect their residents. In 2018, California became the first state to enact a comprehensive privacy law when the California Consumer Protection Act (CCPA) was passed. In 2020, the state amended the CCPA, passing the California Privacy Rights Act (CPRA). In 2021, Virginia followed suit, passing the Consumer Data Protection Act (CDPA). Most recently, in July 2021, Colorado passed the Colorado Privacy Act (CPA). While there are differences, these laws prescribe similar responsibilities for covered entities in an attempt to protect the privacy of consumers.
Several other states have introduced privacy bills, including Florida, Washington state, Texas, Massachusetts, and Nevada. As the number of unique state privacy laws rises, businesses engaged in the buying and selling of consumer data will need to alter their practices in order to remain compliant. Companies that use advertising technology (“ad tech”) to behaviorally target consumers are chief among these, as the digital ad industry’s business model relies heavily on the collection, aggregation, interpretation and disclosure of personal information. Advertisers and internet platforms argue that behavioral ads benefit consumers by fostering a more efficient and personalized web browsing experience, but these practices pose a serious risk to consumer privacy, and have encouraged state lawmakers to address these practices directly. However, state privacy laws still rely largely on a “notice and choice” regulation model, and are not as broad or effective as the public might expect.
This brief will explain the ad tech ecosystem, the various intermediaries involved in the collection, aggregation, and use of consumer data, and the harms associated with these practices. Next, it will explain provisions and themes common among the California, Virginia, and Colorado privacy laws, examine how these laws live up to expectations, and where improvements are needed to properly protect privacy and curb harmful data practices in the ad tech industry.
Editorial disclosure: This brief discusses policies by Amazon, Apple, Facebook, and Google, all of which are funders of work at New America but did not contribute funds directly to the research or writing of this piece. New America is guided by the principles of full transparency, independence, and accessibility in all its activities and partnerships. New America does not engage in research or educational activities directed or influenced in any way by financial supporters. View our full list of donors at www.newamerica.org/our-funding.
Technical Overview
Much of the free internet is supported by the sale of consumer data for advertising. While users are generally aware of this, the mechanisms employed by the ad tech ecosystem remain opaque and complex.
Online advertising campaigns take different forms and generally focus on maximizing interactions with consumers most likely to buy a product, change beliefs, or relate to the content. Publishers benefit from these advertisements as well—relevant content is more likely to keep users engaged for longer periods of time, increasing revenue and control. Contextual advertising is the practice of placing ads based on the content of a page. For example, a cosmetics company would likely seek to place ads on websites geared toward women, since the majority of readers fall into their target audience. In contrast, behavioral advertising, also known as surveillance advertising, “is the practice of extensively tracking and profiling individuals and groups, and then microtargeting, [e.g., precise targeting], ads at them based on their behavioral history, relationships, and identity.” While contextual advertising was previously most common, the evolution of the internet and consumer data collection capabilities has led to the rise of behaviorally targeted advertising. Combined with advanced ad tech and machine learning capabilities, serving precise behavioral advertisements is faster and more scalable than ever before.
Behavioral advertising and contextual advertising transactions begin in similar ways—with a publisher and an advertiser. However, the similarities largely end there. Through a web of interconnected technologies, softwares, servers, and programs, the ad tech ecosystem coordinates the automated purchase and sale of data-driven behavioral advertising on the internet in real time. This process funds many popular social media platforms, search engines, and email accounts and allows these companies to offer free services.
In order to scale the traditional buying and selling of ads to suit the speed of the internet and process large sums of user data, publishers and advertisers rely on multiple intermediaries to facilitate behavioral targeting. These intermediaries—which include demand-side platforms, supply-side platforms, data management platforms, ad servers, ad exchanges, data brokers, and single-site ad platforms—work together to seamlessly exchange and analyze consumer data, determine the most appropriate ad placement, and facilitate ad sales, all in the fraction of a second before the consumer’s web page loads. The result is an advertisement that reflects a user’s supposed preferences and beliefs based on their web browsing activity.
In the ad tech ecosystem, publishers and advertisers exist on opposite ends of the spectrum. On the buy side of the equation, advertisers align with demand-side platforms (DSPs) such as LiveRamp, MediaMath, and Rocket Fuel that manage advertisements and facilitate automatic bidding for ad space with multiple publishers at a time. Advertisers specify targeting criteria and bid prices to their DSPs, which are then used to help make instantaneous decisions about the value of a potential ad space. Often, demand-side platforms rely on data management platforms (DMPs) like Adobe Audience Manager and Salesforce Audience Studio to collect and analyze user data from multiple sources across the web, including data brokers, to curate precise audience segments and determine the most relevant and cost effective ad placement. On the sell side, publishers align with supply-side platforms (SSPs), such as AdColony and Google Ad Manager, which assist publishers in connecting their available inventory to buyers and setting prices, payment terms, and criteria for acceptable advertisers. In comparison to pre-internet advertising, DSPs and SSPs make the process automated, more scalable, and more lucrative on both sides of the transaction. In addition, both the buy and sell sides use ad servers—web servers that work alongside DSPs and SSPs to store and serve advertisements, monitor campaign impressions, and manage inventory.
The bridge between the actors on the buy and sell sides are the ad networks and ad exchanges. Ad networks connect a finite number of publishers through their SSPs to potential buyers. Ad networks are analogous to brokers, connecting groups of buyers and sellers based on need. At the center of these intermediaries, acting as digital trading floors, sit ad exchanges. While technically any entity can buy or sell on ad exchanges, transactions usually flow through ad networks, DSPs, and SSPs. By relying on targeting specifications and user information provided by these entities, ad exchanges facilitate the buying and selling of advertisements at scale.
Data
Data is the lifeblood of the ad tech ecosystem, serving as fuel powering the capability to narrowly target users with personalized ads. While each intermediary serves an instrumental role in the ad tech ecosystem, they would be useless without personal data. User data is derived from a variety of sources, and includes personal information like email addresses and phone numbers, geographic information, engagement data such as page views, clicks, and time spent on a particular page, and attitudinal data, which includes a user's opinions or feelings about a topic. When aggregated, this information has the potential to create highly specific user profiles, which are then loaded on to DSPs, SSPs, or their respective DMPs. This allows players on both the buy and sell side to create specific targeting requirements, and make informed, instantaneous decisions about which consumers would be best suited to be served a particular advertisement. User information is so illustrative that in some instances advertisers engage in one-to-one marketing, delivering a unique ad placement designed for the exact user being targeted.
User data can be categorized in four ways based on who is collecting it and how it is obtained. Zero-party data refers to information that a user actively shares with a website, such as data collected through polls or surveys. Similarly, first-party data is information collected by an advertiser or publisher based on their direct interactions with customers, including subscription information, transaction history, and certain website analytics. Second-party data, relatedly, refers to zero-party or first-party data exchanged between affiliated entities such as business partners. The most contentious category of user information is third-party data—data collected by an entity that does not have a direct relationship with the consumer.
While considered less accurate than zero-party and first-party data, third-party data provides advertisers and publishers convenient access to a large amount of user information. Through tracking cookies, device IDs, location data, IP addresses, and browser fingerprinting, third-party data brokers aggregate mass amounts of consumer information, which they in turn sell to advertisers, publishers, and their supply and demand-side platforms to facilitate the automatic buying and selling of targeted ad space. Despite third-party data’s popularity and instrumentality to the ad tech cycle, its support in the industry is waning. Mozilla Firefox and Apple’s Safari browser both block third-party cookies by default. In 2020, Google announced its intention to remove all third-party cookies from its Chrome browser by early 2022. However, in June 2021, the company extended this date until at least late 2023. While not yet completely extinct, the imminent demise of the third-party cookie on the internet’s most popular platforms will likely prompt the industry to designate more resources to build out robust first-party data sets, relying more heavily on programs discussed below, such as Facebook’s Pixel and Google’s FLoC.
While generally considered to be a “privacy-friendly” alternative to third-party data, first-party and zero-party data still provide detailed information about users who interact directly with a website or brand on the internet that can be incorporated into targeted advertising campaigns. Traditionally, first-party and zero-party data have been considered more accurate and tend to provide consumers with more control over their information. However, as tech giants like Facebook, Google, and Amazon expand their exclusive walled gardens—closed ecosystems that collect, store, and create their own first-party data sets and tools for advertisers—previous assumptions about this data become less accurate.
Facebook and Google, referred to as the “duopoly,” are two of the most frequented sites on the internet, controlling 25.2% and 28.9% of the digital advertising market respectively. With billions of combined users, the duopoly controls two of the most robust first-party data sets. To capitalize on this, Facebook and Google have each developed programs aimed at increasing profits by attracting advertisers and leveraging control over the ad tech market.
Facebook’s Pixel is a first-party cookie advertisers place on their own websites to track a Facebook user’s activity. The cookie sends the user’s activity back to Facebook, where it is added to the site's trove of first-party data and used to inform targeted behavioral ad placements. Since Facebook does not allow advertisers to integrate data from their own DMPs or engage in cookie matching, prospective advertisers have little option but to rely on these data sets. Similarly, Google Ads currently functions as its own opaque ad tech ecosystem, providing advertisers with tools and access to its first-party data sets. Currently, Google provides advertisers with flexibility to use some third-party data, with limits such as a prohibition on using third-party data to create audiences for targeting. This current configuration, however, is poised to change through Google’s newest venture, Federated Learning of Cohorts (FLoC). FLoC is a subsection of Google’s Privacy Sandbox, which will halt traditional cross-site tracking by collecting information directly through users' browsers without cookies and lumping them together in cohorts based broadly on browsing habits, which advertisers can then use to inform ad placements. As the world’s largest web browser, the imminent elimination of third-party cookies promises to change the landscape of behavioral advertising drastically. Google argues this is a positive step that will preserve privacy on the web. Advocates disagree, arguing Google’s cohorts make browser fingerprinting easier and subject users to potential cross-context exposure. While FLoC has not yet been implemented, it is clear that Google’s first-party data will become significantly more important in the future, ultimately leading to even more revenue and control.
When the many pieces of the ad tech ecosystem come together, they deliver personalized content to users’ screens in mere milliseconds. Collaboration begins when a user requests a publisher’s web page from his or her browser. The browser notifies the publisher, and the publisher sends its page to the browser, which usually contains space for advertising content. The publisher immediately gets to work filling its available ad space. It first contacts its ad server, which has ads stored and queued for immediate placement. Based on the information the publisher or its DMP has relating to the user requesting the page, the ad server scans its reserves for a relevant placement. If no relevant ad exists in the server, the publisher contacts the SSP, which sends the user’s information and publisher’s inventory out to the greater ad tech ecosystem; usually to an ad exchange, but also to other ad servers, ad networks, or DSPs. Once the request reaches the ad exchange, the profile of the user loading the ad, in addition to the ad inventory available and price requirements, are sent out to a seemingly limitless number of advertisers through ad networks, DSPs, and ad servers who bid on behalf of advertisers. When the highest bidding advertiser wins the space, its DSP communicates instructions to the publisher’s ad server via the ad exchange and SSP. The publisher’s ad server then forwards the instructions to the browser, which retrieves the content from the winning advertiser’s server. Before the user even has a chance to blink, a highly targeted ad appears on their screen. This is only one example of how advertising technologies work together to deliver personalized content. In light of the varying size, capacity, and need of different ad tech companies and platforms, in conjunction with continued innovation in the space, the precise way a targeted ad makes it to the screen of a user, and the data used to get it there, may vary based on the use of intermediary technologies.
Is Targeting as Lucrative as the Industry Claims?
Claimed Benefits
To justify its data collection practices, the ad tech industry tends to argue that behavioral advertising yields higher click-through rates—the ratio illustrating the frequency with which those served an advertisement actually click on or engage with it—and return on investment, due to the assumption that companies avoid wasting time and ad dollars serving content to uninterested consumers. These high returns, they argue, allow many sites to function without installing paywalls. The industry also claims consumers benefit from seeing ads for items they are likely interested in—reducing search time and providing a more enjoyable experience. Despite these arguments, research suggests that behaviorally targeted ads make only four percent more revenue compared to other types of ad placements. Additionally, other forms of advertising can be more profitable for publishers. For example, in the wake of the EU’s General Data Protection Regulation (GDPR), the New York Times completely switched from behavioral to contextual and geographical advertising in Europe, in addition to blocking open-exchange buying. Following the change, The Times reported, advertising revenue “increased significantly.”
Evidence also suggests that behavioral advertising may not be as accurate as promoted. In fact, a 2019 study found that targeted advertising based on gender was accurate only 42 percent of the time, and targeting based on gender and age only 24 percent of the time. Additionally, considering the opinion of the consumer, another study found users report up to 75 percent of ads served to them are irrelevant.
Potential Harms
In addition to the questionable level of benefit, this collection and use of personal information poses a number of risks. One prominent risk is that of inappropriate discrimination. The core function of behavioral advertising is to discriminate—to differentiate between users with certain preferences and serve them content based on these differences. While it is sometimes innocuous to serve advertisements based on gender, race, or socioeconomic status, this discrimination can also be harmful. Thanks to highly detailed profiles rich with user information, algorithmic ad delivery tools have the potential to exploit these differences and harm vulnerable communities. In 2019, Facebook settled five cases accusing the social media giant and its ad delivery process of allowing housing and employment advertisers to target users inappropriately by race and gender. While the company asserts it has since changed its practices and that advertisers can no longer use Facebook’s advertising platforms for discriminatory housing, employment, or credit ads, loopholes still exist, particularly in application to women and the elderly. Harmful audience categorization that informs targeting also poses a risk, with some platforms suggesting advertisers target users based on factors such as “interested in treason,” “children interested in alcohol,” or based on a user’s political biases and political affiliations.
State Legislation—Is it Making a Difference?
The pervasiveness of data-intensive practices such as behavioral advertising has led to a flurry of legislative action at the state level. As of August 2021, three states—California, Virginia, and Colorado—have passed comprehensive privacy bills, and several more are likely to follow suit. Broadly, the laws impose certain responsibilities on covered entities engaged in the sale of user data, in addition to providing consumers with rights regarding their personal information. The laws also provide methods of redress against companies in violation, either through the enforcement power of state attorneys general or a private right of action for citizens. While some provisions in the trio of laws positively impact consumers and require ad tech companies to alter or reexamine their current practices, others are less effective and do little to reduce the risk associated with the industry’s data collection practices.
Ambiguous Definition of “Sale”
Central to the California Consumer Privacy Act (CCPA), its successor the California Privacy Rights Act (CPRA), Virginia’s Consumer Data Protection Act (CDPA), and the Colorado Privacy Act (CPA) is the broad definition of the “sale” of consumer data. While each uses slightly different language, all define the term more broadly than exchanging money for data. For example, the CCPA defines a sale as “selling, renting, releasing, disclosing, disseminating, making available or transferring a consumer’s personal information by the business to a third party for monetary or other valuable consideration.” CPRA built on the CCPA’s definition, and specifically includes the sharing of information for purposes of cross-context behavioral advertising: “the targeting of advertising to a consumer based on the consumer's personal information obtained from the consumer's activity across businesses.” Colorado and Virginia adopted similar language, but provide key exceptions to the definitions, including transfers to affiliates (second-party data). Additionally, the CDPA leaves out the vague language “or other valuable consideration” present in CCPA, CPRA, and CPA.
This variation and vagueness causes general consumer confusion and allows for inconsistent data handling practices. Since much of the behavioral advertising process involves exchanging user data fluidly, and doesn’t necessarily require a direct exchange of monetary consideration among parties, companies may view the requirements of the laws differently depending on their subjective interpretation. The use of terms such as “other valuable consideration” in CCPA, CPRA, and CPA also creates ambiguity, leaving the safety of consumer data at the hands of companies who must determine if their practices constitute a sale absent substantial regulatory direction. Additionally, which relationships constitute an affiliation for purposes of CDPA and CPA is also unclear. The lack of clarity has the potential to lead to compliance difficulties, and may end up doing more harm than good for consumers. As courts begin litigating complaints pursuant to these laws and attorneys general begin exercising authority to clarify definitions, a better understanding of what exactly constitutes a sale will inevitably emerge. Until then, however, the full scope of these laws remains vague.
Data Protection Assessment Requirements
CPRA, CDPA, and CPA all require data controllers to engage in certain privacy audit requirements. In amending the CCPA, CPRA created a Consumer Privacy Protection Agency (CPPA) to which covered entities whose practices impose “significant risk” to user privacy must submit risk assessments. In determining when risk is significant, the law points to factors such as the size and complexity of the business, along with the nature of the processing activities. These risk assessments, which must be filed with the CPPA on a regular basis, require the covered business to identify whether their practices involve processing sensitive personal information and weigh the benefits against the potential risks of the processing. While CPA and CDPA do not establish designated privacy agencies, they also impose data protection assessments on covered entities that specifically require businesses engaged in targeted advertising to conduct audits explaining the risks and benefits associated with the profiling. These assessments are reviewed by the state’s respective attorney general.
Data protection assessment requirements impose a few notable obligations on the ad tech industry. First, since the CPRA’s definition of “significant risk” is broad, covered businesses engaged in behavioral advertising may be unsure what exactly their responsibilities are under the law and how these obligations vary from those imposed under the GDPR’s Data Privacy Impact Assessment. As with the definition of sale, the true meaning of this provision will become clearer as the issue is litigated in California courts, but this will likely take time. For ad tech companies subject to CDPA and CPA, the applicability of these provisions is clearer, but they will still be required to ensure they are complying with the detailed requirements involved in completing the required assessments, including keeping copious records of tracking activity and identifying potential risks clearly. While the required data protection assessments will be privileged and not automatically available to the public, they will still provide consumers with tangible benefits. Notably, risk assessments will help promote industry transparency with state government, and aid in the investigatory and enforcement processes when a covered entity violates the law.
Opt-Out Requirements
California, Virginia, and Colorado all require covered entities to provide a clear and conspicuous method for consumers to opt out of the sale of their personal data, with Virginia and Colorado specifically requiring targeted advertisers to do so. Additionally, Colorado goes even further, requiring that companies engaged in targeted advertising provide a user-selected universal opt-out mechanism.
Critics argue that while these opt-out requirements impose some additional burdens on the ad tech industry and create the illusion of consumer privacy, they do not go far enough to properly protect citizens. Primarily, privacy advocates argue that an opt-out regime such as the ones established in CCPA, CPRA, CDPA, and CPA shift the onus to the consumer and relieve data controllers of too much responsibility, making privacy an option rather than the default approach. Additionally, while a universal opt-out mechanism is a step in the right direction and provides users with an accessible way to prevent online tracking, the CPA’s provision only applies to information sold for behavioral advertising purposes. This means that even users who select a universal opt-out option may still be subject to intrusive, unnecessary tracking resulting in a false sense of security.
Controller Duties
In addition to data protection assessments, CPA also mandates that data controllers, including those engaged in targeted advertising, adhere to certain duties. These include the duty of care, duty of transparency, duty of data minimization, duty to avoid secondary use, duty to avoid unlawful discrimination, and and duty to obtain consumer consent before processing sensitive data. The CPA’s requirements generally track the Fair Information Practice Principles (FIPPs) promoted by privacy advocates globally, emphasizing consumer control of information and professional responsibility. On paper, these duties certainly seem to be a step in the right direction, providing more protection for consumers and placing greater responsibility on industry. However, only time will tell if Colorado’s Attorney General will be able to enforce them in a meaningful way.
The Right to Cure
A “right to cure” is an opportunity for an at-fault party to remedy their violation of a statute or contract before enforcement action is taken. Under the CDPA and CPA, businesses are given a 30-day and 60-day cure window, respectively. The CPA’s cure window, however, will be phased out by 2025. Previously, the CCPA also provided a 30-day cure period—however, the CPRA will eliminate this when it officially takes effect in 2023, instead providing the CPPA discretionary authority to allow violators to cure on a case-by-case basis.
Right-to-cure provisions do not provide consumers with more protections for their personal privacy, and without meaningful oversight, it may be difficult to ensure offenders actually remain in compliance after taking the opportunity to correct their practices. Privacy advocates argue cure provisions contradict the point of regulation in the first place and are too lackadaisical on offenders, allowing companies to actively evade compliance unless they are caught. However, in California and Colorado, where the right to cure will be eventually phased out, covered entities may be incentivized to adjust their practices promptly in order to avoid incurring civil penalties. What’s more, providing a cure period that will be phased out over time is helpful because it allows covered entities to experiment and adjust their practices through trial and error, creating a window to develop compliance strategies that fit best with their business models without fear of penalty for mistakes made while adjusting.
Dark Patterns Prohibition
Dark patterns are “digital tricks” defined as "a user interface designed or manipulated with the substantial effect of subverting or impairing user autonomy, decision-making, or choice, as further defined by regulation." For example, in extreme uses of dark patterns, a malicious actor may display a mobile advertisement with what appears to be a speck of dirt, or a scratch on the users screen in an attempt to manipulate them into tapping the banner, releasing a virus or malicious code. Dark patterns are also used by legitimate businesses through careful wording and deceptive web design. Recently, companies engaged in targeted advertising have reportedly engaged in the use of dark patterns such as misleading or confusing phrasing or web design to push users toward accepting weak privacy settings, or consenting to the sale of their data. To combat this practice, CPRA and CPA both explicitly provide that consent acquired through the use of dark patterns is a violation of the law.
For ad tech companies covered under CPRA and CPA, the dark patterns prohibition will likely not make much of an impact, aside from prompting companies to pay closer attention to how they present users with options and ask for consent. On the other hand, prohibiting dark patterns doesn’t do much to advance consumer privacy, either. Opt-out consent regimes are disfavored by privacy advocates due to the inherent expectation that consumers alone are responsible for protecting their personal information. Prohibiting dark patterns doesn’t fix this problem—it simply acts as a Band-Aid. If lawmakers advanced a “privacy by default” standard, rather than passing the onus to consumers, there would be little need for a prohibition on dark patterns at all.
What’s Missing?
Though the flurry of action at the state level has led to some positive legislative outcomes, the current laws in California, Virginia, and Colorado fail to provide for some extremely important safeguards, such as biometric privacy protections and data minimization principles, and lack creativity in their approaches to protecting privacy. Broadly, these three laws focus their efforts on providing users with means to control their data, without placing the onus on industry. A 2020 Consumer Reports study found that with so much responsibility on consumers, the seemingly positive provisions in the CCPA were failing to adequately regulate data collection. The report also noted that consumers struggle to locate the “Do Not Sell” link as required by the CCPA, and often are unsure whether their attempt to opt out was successful. The trend among state lawmakers to give citizens “control” of their data seemingly does little to help consumers, who continue to struggle to exercise their rights and remain at risk. Moving forward, states looking to pass comprehensive privacy laws should attempt to break free of the current mold, incorporating meaningful provisions to advance the goal of privacy by design.
Conclusion
Despite the hurdles created by state privacy legislation, the biggest nuisance for players in the ad tech ecosystem may be establishing a compliance program that can address all of them. The scale and interconnectivity of the ad tech industry makes it likely that a company subject to compliance in one state is also subject to compliance in another, meaning these organizations must be privy to the nuances of each. While not detrimental to the business model, the differing jurisdictional requirements may result in varying protection for users based on their residence. The solution is federal privacy legislation, but Congress has had difficulty advancing a bill. Additionally, it remains unclear how effective state privacy legislation will be in regulating the rapidly changing ad tech industry moving forward. As walled gardens and first-party data collection practices become more prominent, and programs such as Google’s FLoC take hold, the usefulness of current laws may be lost and legislation may need to adapt to regulate more effectively.
With so much uncertainty in the industry and a partisan stalemate at the federal level, it is imperative state lawmakers focus on crafting privacy laws that shift the burden from consumers to industry. Companies engaged in behavioral advertising should be held accountable for their data handling practices through provisions that promote and encourage transparency, stricter corporate obligations, user rights and controls, and privacy by default.