The Moral Gray Space of AI Decisions
Blog Post
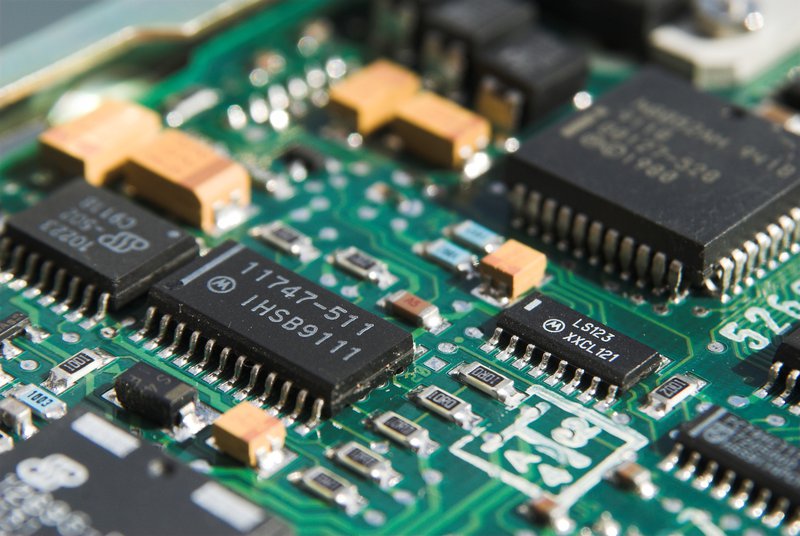
Dec. 13, 2018
This is part of The Ethical Machine: Big ideas for designing fairer AI and algorithms, an on-going series about AI and ethics, curated by Dipayan Ghosh, a former Public Interest Technology fellow. You can see the full series on the Harvard Shorenstein Center website.
PATRICK LIN
PROFESSOR OF PHILOSOPHY AND DIRECTOR, ETHICS + EMERGING SCIENCES GROUP, CALIFORNIA POLYTECHNIC STATE UNIVERSITY, SAN LUIS OBISPO
Artificial intelligence in diverse applications—from sex bots to war machines—is giving rise to equally diverse concerns: algorithmic bias, transparency, accountability, privacy, psychological impact, trust, and beyond. Of course, all of these issues don’t necessarily arise in all forms of AI; for instance, few people, if any, care about privacy with military robots. But one root ethical issue that does apply to the entire technology category is the general ability to make decisions. This is the linchpin issue to be examined here.
While there’s no consensus around how to define “artificial intelligence” or even “intelligence,” here’s a working definition: AI is a computational system designed to automate decisions, with the appearance of intelligence. A robot, then, is embodied AI that translates those decisions into physical tasks. Given that a core function of artificial intelligence is to automate decisions, it’s fair to ask whether AI fulfills this function “correctly.”
The question is more difficult than it may appear once we recognize that there are three kinds of AI decisions. First, there are “right” ones, that is, uncontroversial decisions that are made as intended or expected (putting aside the question of whether they’re objectively right; the point is that no one has a real problem with them). Second, there are wrong decisions, such as when an undesired outcome is caused by bad design (e.g., programming errors) [1], or by emergent behavior (e.g., “flash crashes” that unexpectedly arise from the sheer complexity of the system or interacting programs) [2], or by “gaming” an AI system with adversarial examples (i.e., inputs that intentionally cause it to make a mistake) [3]. But, third, there are also decisions that are neither obviously right nor wrong, as they fall within the shadowy but sizable space of judgment calls.
Those judgment calls, crystallized as code, are the ones that demand serious ethical consideration, especially since they may raise challenges to risk and liability.
Those judgment calls, crystallized as code, are the ones that demand serious ethical consideration, especially since they may raise challenges to risk and liability. If not considered with due care, this moral gray space could both derail technological progress and harm things that matter, such as inappropriately denying a job to someone who does not fit the profile of what a programmer or system thinks is a good applicant.
Case Study 1: Autonomous Cars
Let’s look at self-driving cars as a case study that hold lessons for other types of AI [4], especially since they’re poised to be the first robotics integrated into society at scale, setting the tone for other autonomous systems that follow. In an unexpected driving emergency, a bad decision by a human driver—say, swerving into another car—could be forgiven as a panicked reflex without forethought or malice. But the same action by a self-driving car, no matter how reasonable, would be scripted or otherwise predetermined, even if the action was learned (e.g., via neural networks). Either way, the AI decision is less like an innocent accident and more like premeditated injury; and both ethics and law treat these very differently, the second much more harshly.
Admittedly, crash dilemmas trigger an allergic reaction in some people who complain they’re too fake. This is perhaps best represented by the infamous trolley problem, a dilemma that imagines a no-win choice between switching the tracks of a speeding train which would then kill one person instead of the five others who are initially in its way, or doing nothing and letting the five people die [5]. Though that popular criticism really misses the point [6], we can also find automated decisions in everyday scenarios, if you want more realism.
Consider a common situation where a self-driving car is driving in the middle lane of a highway [7]. If it’s flanked by a large truck on one side but a small car on the other side, where should the robot car be positioned within its own lane? There’s no obviously right answer here. It’d be reasonable to instruct the car to stay exactly in the middle of its lane, if there’s no actual emergency; or to give wider berth to the truck, even if it means nudging closer to a smaller car; or to even make the opposite decision and give more room to the smaller object, as the most vulnerable in the scenario. (This last decision can be made clearer by imagining the smaller object were a motorcyclist, bicyclist, or pedestrian.)
Judgments about risk are often unstated assumptions and not proactively defended.
All of the above answers are defensible, but those judgments about risk are often unstated assumptions and not proactively defended. If we can’t see how the moral math is being worked out, then it’s unclear that AI developers have done their due diligence and taken proper care in designing their products. Giving preferential treatment to one class of objects, such as giving wider berth to trucks, transfers some amount of risk to other road users without their consent or even awareness [8]. This and other design choices, as reasonable as they seem, may hold legal implications to industry’s surprise.
Though it might sound ordinary, lane positioning is actually a safety-critical decision that can share the same kind of trade-offs found in more dramatic crash scenarios. Any programming decision that involves a trade-off—such as striking object x instead of y, or increasing distance away from x and toward y—requires a judgment about the wisdom of the trade-off, that is, about the relative weights of x and y. AI decisions are generally opaque to most of us already, but safety-critical AI decisions, including risk-benefit calculations, demand special attention and transparency.
Case Study 2: Traffic-Routing Apps
As an AI technology deployed widely today and again with relevance for other forms of AI, let’s look at the traffic-navigation app Waze to draw out the hidden ethics in more everyday scenarios. Often, there’s more than one reasonable way to get to a destination: one route could be the shortest distance but involve heavier traffic, or another may be lengthier but faster, or the longer route may be more scenic, and so on. These might not seem ethically problematic, until we realize that route selection involves risk. For instance, the fastest route may be more dangerous statistically if it includes more intersections, left turns, pedestrians, and other risk factors.
Apps such as Waze will generally default to the fastest route even if it’s statistically dodgier [9]. This creates possible liability for making that dangerous choice without the user’s consent or knowledge, especially if the decision leads to an accident. But there’s also liability in ignoring risk data that’s readily available, such as insurance and government statistics on where the most accidents occur in a certain town. Waze is also giving rise to complaints about “flocking” behavior: groups of cars are sent by algorithms through quiet neighborhoods not designed for heavy traffic [10]. This could increase risk to children playing on these streets, lower property values because of the added road noise, and create other externalities or unintended harms.
But let’s suppose Waze wants to account for that risk data: Should it avoid poorer neighborhoods if there’s a statistical risk for increased crime or accidents? Any answer will be controversial. Even if crime data identifies these areas as clear risks, it could still be discriminatory to route traffic around them. For instance, related to structural racism, over-policing in minority communities can generate more incident reports and data, and this makes their situation look worse than it really is [11]. Routing around those neighborhoods could also harm local merchants who’d then be less visible to potential customers, further aggravating the economic depression that already tends to exist in such areas.
On the other hand, if certain data about neighborhoods is excluded in decision-making for the sake of equality—such as median income—then liability is created if there were a correlation between risk and that data. Again, the base ethical dilemma here of making one choice to the detriment of something else resembles the difficult trade-off required in the trolley problem and other “fake” crash dilemmas. Indeed, given their large-scale effects, these everyday scenarios suggest that technology developers are actually unwittingly crafting public policy—a serious activity that clearly demands all of your wits.
Relevance to Other AI Systems
The case studies offered above are versatile; their general lessons can be applied to other AI areas. To start with, there’s a natural link here to ground and aerial delivery drones, which also need to navigate through social spaces [12]. This means making decisions related to human interaction, some of them safety-critical in nature. Social robots, such as “care bots,” also may need to make judgment calls—decisions that are neither obviously right nor wrong—that weigh a patient’s autonomy against their well-being or doctor’s orders (e.g., if they refuse to take their medication) [13].
In AI decision-making across the spectrum, we need to be made aware of the assumptions, biases, and background considerations that invisibly power our technologies.
In AI decision-making across the spectrum, we need to be made aware of the assumptions, biases, and background considerations that invisibly power our technologies. Without that, we cannot hope to understand the risks and therefore cannot make informed decisions. At the same time, technology developers must tread carefully and transparently in this moral gray space: liability and trust implications can be contained for only so long under the cover of intellectual property and trade secrets before they blow up. As AI takes over more of our jobs, it also takes on new responsibilities and duties, perhaps more than technology developers appreciate today. Thinking openly about ethics now is crucial to their survival—as well as to ours.
References
- Patrick Lin, “Here’s How Tesla Solves a Self-Driving Crash Dilemma,” Forbes, April 5, 2017, http://www.forbes.com/sites/patricklin/2017/04/05/heres-how-tesla-solves-a-self-driving-crash-dilemma/.
- Drew Harwell, “A Down Day on the Markets? Analysts Say Blame the Machines,” The Washington Post, February 6, 2018, https://www.washingtonpost.com/news/the-switch/wp/2018/02/06/algorithms-just-made-a-couple-crazy-trading-days-that-much-crazier/.
- OpenAI, “Attacking Machine Learning with Adversarial Examples,” OpenAI blog, February 24, 2017, https://blog.openai.com/adversarial-example-research/.
- Patrick Lin, “The Ethical Dilemma of Self-Driving Cars,” TED-Ed, December 8, 2015, https://www.youtube.com/watch?v=ixIoDYVfKA0.
- Lauren Davis, “Would You Pull the Trolley Switch? Does It Matter?” The Atlantic, October 9, 2015, https://www.theatlantic.com/technology/archive/2015/10/trolley-problem-history-psychology-morality-driverless-cars/409732/.
- Patrick Lin, “Robot Cars and Fake Ethical Dilemmas,” Forbes, April 3, 2017, https://www.forbes.com/sites/patricklin/2017/04/03/robot-cars-and-fake-ethical-dilemmas/.
- Noah Goodall, “Away from Trolley Problems and Toward Risk Management,” Applied Artificial Intelligence 30, no. 8 (2016): 810–821, https://www.tandfonline.com/doi/full/10.1080/08839514.2016.1229922.
- Patrick Lin, “The Robot Car of Tomorrow Might Just Be Programmed to Hit You,” Wired, May 6, 2014, http://www.wired.com/2014/05/the-robot-car-of-tomorrow-might-just-be-programmed-to-hit-you/.
- Linda Poon, “Waze Puts Safety Over Speed by Minimizing Left Turns in LA,” CityLab, June 20, 2016, https://www.citylab.com/life/2016/06/waze-puts-safety-over-speed-by-minimizing-left-turns-in-la/487577/.
- John Battelle, “The Waze Effect: AI and the Public Commons,” Newco Shift, February 3, 2016, https://shift.newco.co/the-waze-effect-ai-the-public-commons-d3926fce108e.
- David Kennedy, “Black Communities: Overpoliced for Petty Crimes, Ignored for Major Ones,” Los Angeles Times, April 10, 2015, http://www.latimes.com/opinion/bookclub/la-reading-los-angeles-kennedy-ghettoside-20150404-story.html.
- Sibil Nicholson, “Latest Amazon Patent Includes Gesture-Recognizing Drones,” Interesting Engineering, March 25, 2018, https://interestingengineering.com/latest-amazon-patent-includes-gesture-recognizing-drones.
- Michael Anderson and Susan Anderson, “Machine Ethics: Creating an Ethical Intelligent Agent,” AI Magazine 1, no. 28 (2007): 15–26, https://www.aaai.org/ojs/index.php/aimagazine/article/view/2065.