Don’t Believe Every AI You See
Blog Post
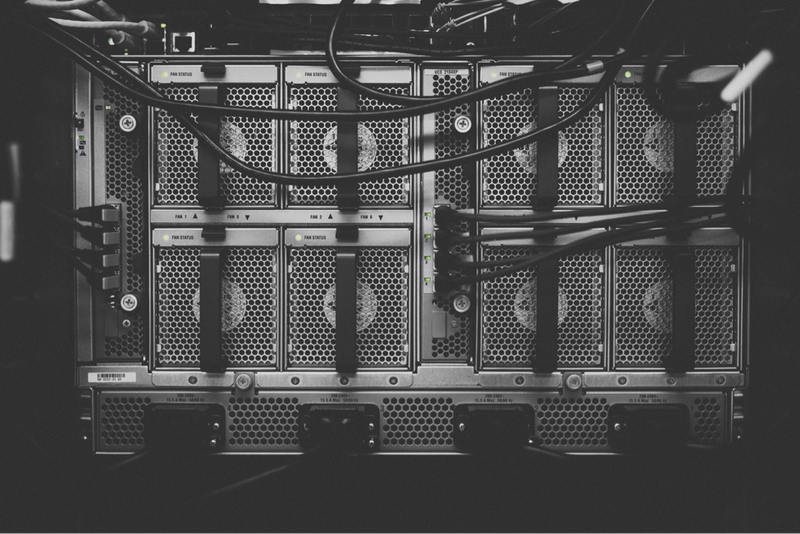
Nov. 26, 2018
This is part of The Ethical Machine: Big ideas for designing fairer AI and algorithms, an on-going series about AI and ethics, curated by Dipayan Ghosh, a former Public Interest Technology fellow. You can see the full series on the Harvard Shorenstein Center website.
M.C. ELISH
RESEARCH LEAD, DATA & SOCIETY RESEARCH INSTITUTE
DANAH BOYD
FOUNDER AND PRESIDENT, DATA & SOCIETY RESEARCH INSTITUTE; PRINCIPAL RESEARCHER, MICROSOFT RESEARCH; AND VISITING PROFESSOR AT NEW YORK UNIVERSITY
At a recent machine learning conference, Ali Rahimi—a leading research scientist at Google— sparked a controversy. During his acceptance speech after receiving the “Test of Time” award honoring a lasting contribution to the field, Rahimi provocatively proposed that “machine learning has become alchemy.” He argued that even though alchemy “worked,” it was based on unverifiable theories, many of which turned out to be false, such as curing illness with leaches or transmuting metal into gold. The parallel is that many of today’s machine learning models, especially those that involve the use of neural networks or deep learning, are poorly understood and under-theorized. They seem to work, and that is enough. While this may not matter in every instance, Rahimi emphasized, it is profoundly consequential when it comes to systems that serve important social functions, in fields such as healthcare and criminal justice, and in tasks like determining credit worthiness and curating news. He concluded: “I would like to live in a world whose systems are built on rigorous, reliable, verifiable knowledge, and not on alchemy.”
Rahimi’s critique and incredulity stands in direct contrast to a prevailing rhetoric around AI and machine learning, which presents artificial intelligence as the apex of efficiency, insight, and disinterested analysis. And yet, AI is not, and will not be, perfect. To think of it as such obscures the fact that AI technologies are the products of particular decisions made by people within complex organizations. AI technologies are never neutral and always encode specific social values.
And yet, AI is not, and will not be, perfect. To think of it as such obscures the fact that AI technologies are the products of particular decisions made by people within complex organizations.
Still, for most of the public, AI feels like magic. That is not accidental. Companies such as IBM, Google, and Amazon shape public understandings of AI—and sell products—through media spectacle. From a well-publicized, six-game match in which IBM’s Deep Blue algorithm beat the world chess champion in 1997, to Google’s AlphaGo program defeating the reigning champion of Go—an abstract strategy game that was long considered harder for a computer to win than chess—hyped marketing events are meant to signal unprecedented advances in computing. Big tech companies also sell the story that better and more sophisticated AI is just around the corner, playing into popular media narratives that equate human and machine intelligence, from The Jetsons and 2001: A Space Odyssey to Her and Westworld. In tackling games that are seen as central to human cognition, performative AI systems give the impression that there’s not a big gap between IBM Watson and “Data,” the humanoid android on the Star Trek television series. But there is. One is designed to perform a discrete task and trained on a specific set of data. The other is an autonomous being able to participate in society.
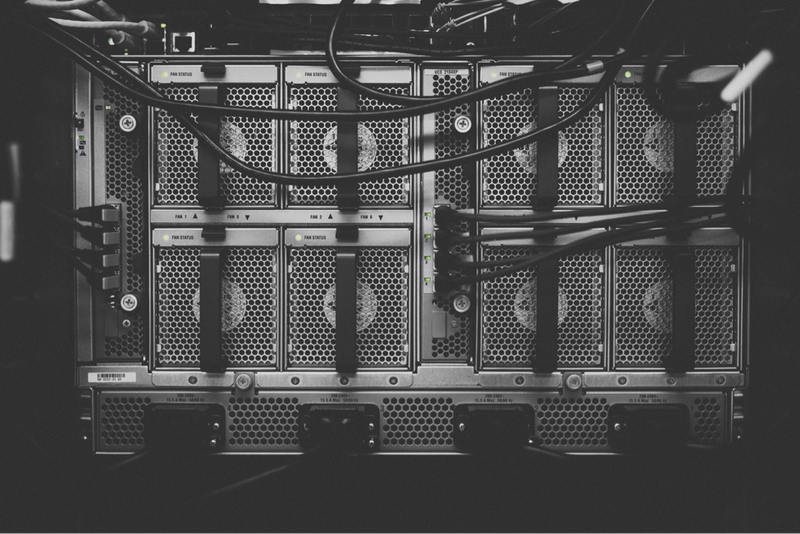
When we consider the ethical dimensions of AI deployments, in nearly every instance the imagined capacity of a technology does not match up with current reality. As a result, public conversations about ethics and AI often focus on hypothetical extremes, like whether or not an AI system might kill someone, rather than current ethical dilemmas that need to be faced here and now. The real questions of AI ethics sit in the mundane rather than the spectacular. They emerge at the intersections between a technology and the social context of everyday life, including how small decisions in the design and implementation of AI can create ripple effects with unintended consequences.
As we think about new configurations for AI and ethics, we need to question not only what AI systems do, but also how they do it. The current machine learning-driven advances in AI are based on the vast quantities of data and processing power that have developed in recent years. In other words, the “smartness” of AI comes from a system’s ability to process and analyze huge amounts of data, beyond the scale of any individual human, in order to predict or automate certain activities such as who is more likely to repay a loan or which search result is likely to be most relevant. The datasets and models used in these systems are not objective representations of reality. They are the culmination of particular tools, people, and power structures that foreground one way of seeing or judging over another. Without comprehensively accounting for the strengths and weaknesses of technical practices, the work of ethics—which includes weighing the risks and benefits and potential consequences of an AI system—will be incomplete.
Digging into the details is where the work of ethically assessing AI technologies begins. Here, we offer three questions to surface everyday ethical challenges raised by AI:
- What are the unintended consequences of designing systems at scale based on existing patterns in society?
The intelligence of AI promises to provide insight into the future, but its predictions are drawn from the past. This means that existing prejudices or structural inequalities may be not only reproduced, but also amplified. Consider, for example, the various technologies involved in offering up an advertisement based on a search query. Search engines integrate an array of data, including personal web history, data obtained by data brokers, clicks by other people on that query, the content of the query, and so on, to produce a model of what an individual might value and click on. But how should we contend with what happens when cultural prejudices are integrated into that system?
Computer scientist Latanya Sweeney has conducted research that demonstrates exactly what can happen, with disturbing ramifications. Her study began when she Google-searched her own name. What came back to her when she did were ads suggestive of an arrest record. (She didn’t have one.) Curious, she decided to experiment with common names given to black and white babies and test how an anonymized user search on Google would affect the ads shown. To her frustration, characteristically black names produced ads implying a criminal record when characteristically white names did not. What is to be gleaned from this research is not that Google is racist through any explicit intention. Rather, Google’s algorithms were optimizing for the racially discriminating patterns of past users who had clicked on these ads, learning the racist preferences of some users and feeding them back to everyone else. In other words, Google learned how to be racist and applied what it had learned to all of its users.
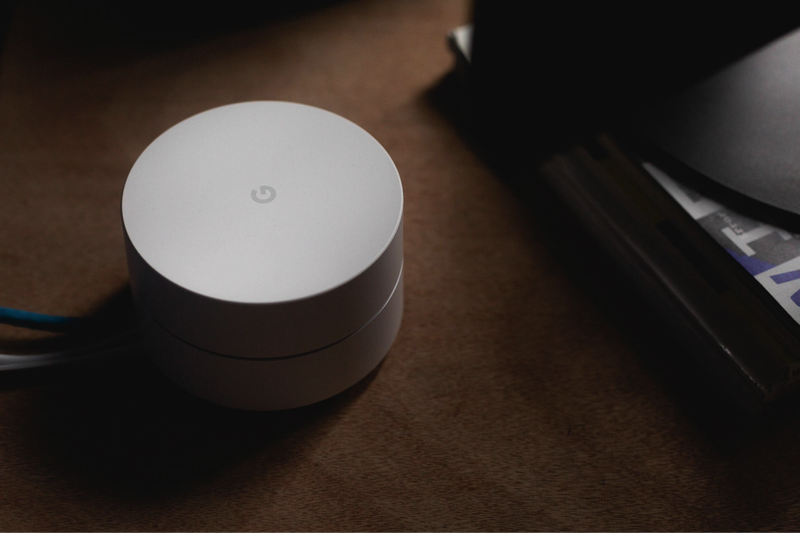
This magnification of prejudice introduces a basic conundrum for those using data to build AI systems. Should AI designers try to remedy cultural prejudices or allow their systems to represent, and thereby reinforce, those prejudices? The former isn’t easy, in no small part because AI technologies are unable to assess values or norms without being explicitly told to look out for them. The latter relies on prevailing popular opinion to establish normative values, often harming marginalized or minority voices. Either choice presents significant ethical trade-offs, and neither can be resolved through an algorithm alone.
- When and how should AI systems prioritize individuals over society, and vice versa?
A primary functionality of AI systems is the ability to produce highly personalized recommendations based on immense aggregated data. Paradoxically, this can put a public good in tension with an individual good. For example, the use of AI systems to improve public health may come at the cost of individual privacy, a tension at the heart of a public controversy involving an automated health app developed by DeepMind, an AI company owned by Google. Ambitiously designed in collaboration with the UK’s National Health Service to help analyze test results and determine treatment, the app relied on 1.6 million patient records provided by London’s Royal Free Hospital illegally. How are these two reasonable goals—improving healthcare and protecting people’s privacy—to be evaluated?
In another case altogether, let’s think about search engines. When looking for content, most individual users don’t want paternalistic AI systems. They want to be given what they want, regardless of how deeply flawed it is. Climate deniers don’t want to see information that denies their worldview any more than feminists do. Since, in most instances, the AI underlying search engines is designed to maximize for user desires, there’s a high likelihood that the current systems only reinforce cultural and ideological divisions.
- When is introducing an AI system the right answer—and when is it not?
We’ve seen many missteps and failures come from trying to solve a social problem with a technical solution. This points toward a third ethical challenge more basic than those above: when and where is it irresponsible to introduce AI systems in the first place? Consider, for example, the domain of judicial decision-making, a highly contested area of criminal justice wherein relatively simple—but proprietary—machine learning systems produce scores to assess someone’s risk of future criminal activity. There is no consensus about whether or not introducing these scores into the judicial decision-making process is responsible.
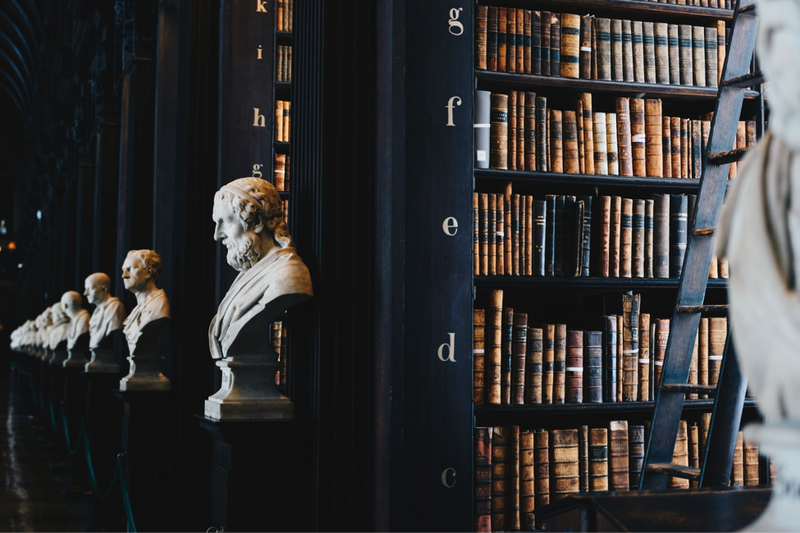
Although the idea of efficiency plays a role here, most conversations focus on whether or not the scores are unfairly discriminatory, or instead actually create a form of fairness or neutrality that is currently missing inside judicial decision-making, where prejudice and bias are commonplace. From a technology-centric point of view, accuracy and mathematically defined constructions of fairness are constraints to optimize for, which makes them highly desirable. But while many advocates and practitioners debate over the right construction of fairness, perhaps an even more fundamental challenge in this case is the larger lack of consensus around the goal of criminal justice punishment—let alone what is “fair.” For some, punishment is the end in itself. For others, the purpose is rehabilitation or a signal of deterrence. If we can’t even answer this basic question that shapes the data, is it ethical to expect a technology to assess future criminal activity and then codify these assessments in a black-box system? Or, from a product design perspective, how can we assess the achievements or consequences of a technology if there is ongoing disagreement about the very metrics of success? After all, those who believe that criminal justice is supposed to rehabilitate see a risk metric as an indicator of a failed system, not a flawed person. From such a viewpoint, perfecting a measure of a person is both counterproductive and cruel.
The invocation of ethics has no doubt begun to emerge alongside AI as its own kind of buzzword. It is easy to gloss over the need to hash out normative ideas within society, challenge what is taken for granted, and question whether or not the approaches we are taking are fair, just, and responsible. Unfortunately, ethically assessing technology cannot be reduced to a one-size-fits-all checklist. When it comes to AI and ethics, we need to create more robust processes to ask hard questions of the systems we’re building and implementing. In a climate where popular cultural narratives dominate the public imaginary and present these systems as magical cure-alls, it can be hard to grapple with the more nuanced questions that AI presents. Ethical debates based on hypothetical or fictitious AI technologies of the far future more often than not just create unnecessary distractions. AI is at a crossroads, and now is the time to lay the foundation for the beneficial and just integration of these technologies into society. This starts by making certain that we’re seeing AI for what it is, not just what we hope or fear it might become.