Algorithms Couldn't Predict How the Pandemic Would Affect Our Lives
Article In The Thread
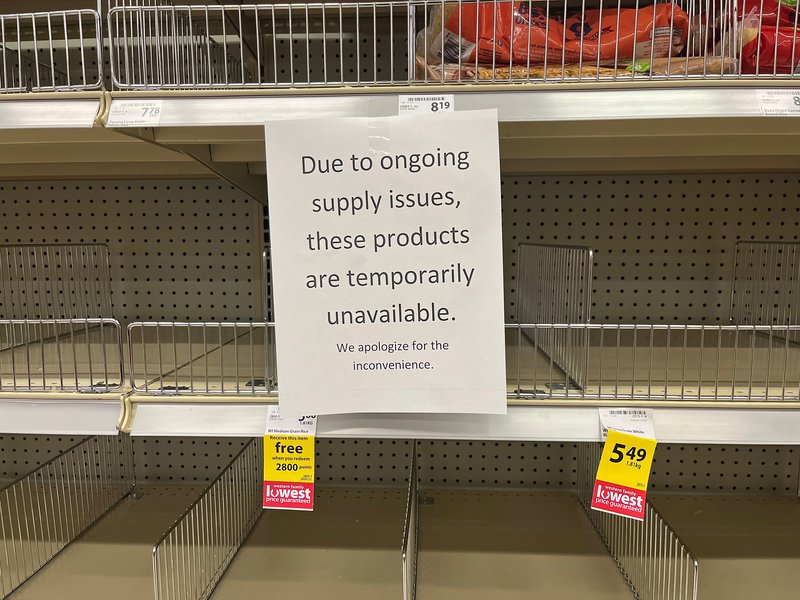
Jenari / Shutterstock.com
May 10, 2022
Algorithms have always had some trouble getting things right — hence the fact that ads often follow you around the internet for something you’ve already purchased.
But since COVID upended our lives, more of these algorithms have misfired, harming millions of Americans and widening existing financial and health disparities facing marginalized groups. At times, this was because we humans weren’t using the algorithms correctly. More often it was because COVID changed life in a way that made the algorithms malfunction.
Take, for instance, an algorithm used by dozens of hospitals in the United States to identify patients with sepsis — a life-threatening consequence of infection. It was supposed to help doctors speed up transfer to the intensive care unit. But starting in spring of 2020, the patients that showed up to the hospital suddenly changed due to COVID. Many of the variables that went into the algorithm — oxygen levels, age, comorbid conditions — were completely different during the pandemic. So the algorithm couldn’t effectively discern sicker from healthier patients, and consequently it flagged more than twice as many patients as “sick” even though hospital capacity was 35 percent lower than normal. The result was presumably more instances of doctors and nurses being summoned to the patient bedside. It’s possible all of these alerts were necessary — after all, more patients were sick. However, it’s also possible that many of these alerts were false alarms because the type of patients showing up to the hospital were different. Either way, this threatened to overwhelm physicians and hospitals. This “alert overload” was discovered months into the pandemic and led the University of Michigan health system to shut down its use of the algorithm.
We saw a similar issue first-hand in the hospital where we both work: We recently published a study examining a health care machine-learning algorithm used to identify the sickest of patients with cancer. Flagging them gives clinicians an opportunity to talk to them about their preferences for end-of-life care. Our data showed that, during the pandemic, this algorithm was 30 percent less likely to correctly identify a sick patient who needed such a timely conversation. Missed end-of-life conversations often translate to unnecessary treatments, hospitalizations, and worse quality of life for individuals who would have instead benefited from early hospice care.
In another example, American Express designed a complex AI algorithm to detect fraud that had 30 percent better performance than its legacy algorithms. However, starting in March 2020, consumers made massive changes in spending patterns due to the pandemic, including larger purchases, more online orders, and many new customers showing up at department stores to buy items like toilet paper and hand sanitizer. Luckily, Amex did some pre-rollout testing and found that this sea change would have triggered an inordinate number of fraud alerts, forcing the company to delay rollout of the algorithm by nearly a year.
The banking sector was the biggest investor in AI prior to the pandemic, as it may help to set more accurate mortgage or interest rates. However, patterns of in-person and online banking changed dramatically during the pandemic. In a Bank of England survey, more than one-third of banks reported that their predictive algorithms became more inaccurate during the pandemic. This has translated to an expected decrease in the pace of AI investment by banks.
How is it that COVID infected our algorithms? The answers are subtle, but offer important lessons since the COVID era will likely impact algorithms for years to come.
First, algorithms do best at pattern recognition. They are usually designed using years of historical data to predict outcomes in the future. However, nearly every input into AI algorithms changed during COVID. In health care, for example, cancer screenings, doctor’s visits, and elective surgeries declined dramatically and still haven’t fully recovered. A pre-COVID algorithm may have predicted that individuals who didn’t see the doctor too often were healthy. But during COVID, sicker patients often avoided the hospital or doctor’s office. Sometimes they got care delivered to them in their homes by outside entities. More often they just didn’t receive care at all. Because of this decreased use of health care services, sicker patients did not have as much data to contribute to predictive algorithms. And thus, algorithms during the pandemic likely under-identified these sicker patients.
Second, the outcomes that algorithms predict changed dramatically during COVID. Take, for example, an algorithm that predicts a patient’s risk of dying. While the algorithm may have been accurate at predicting death prior to COVID, the rate of death across the country increased by 40 percent between late 2019 and late 2020. The underlying relationships between risk factors and outcomes changed dramatically. So, algorithms can malfunction when the frequency of an outcome like death changes so much in such a short amount of time.
Third, COVID’s impact on health care and spending habits were particularly stark for marginalized populations, and that has led to algorithms being more likely to misfire for poor and nonwhite individuals. Prior to COVID, nonwhite and low-income Americans were significantly more likely to pay cash in a store rather than shop online. Fast-forward to the pandemic, where all segments of the U.S. population shifted from brick-and-mortar stores to online purchasing. A fraud detection algorithm may have been more likely to flag purchases from low-income individuals and minorities who seemingly suddenly changed their purchasing patterns toward more online shopping.
The pandemic has compromised our algorithms. But there are ways to fix this problem — and prevent it from happening again.
First, humans should exercise greater oversight over AI algorithms — at least for the time being. Any organization that uses pre-COVID AI algorithms should double-check their performance, particularly for how they are affecting marginalized groups like Black Americans and other minorities.
Second, if these checks reveal any red flags, organizations should redevelop (or “retrain”) their algorithms using data from the pandemic era. This is particularly relevant for algorithms that use inputs that are still affected by COVID.
Third, we need to develop algorithms that are robust to future disruption. Novel AI techniques may be able to “self-learn” during different crises. During the pandemic, a reinforcement learning algorithm used by border control agencies in Greece successfully limited the influx of asymptomatic travelers infected with COVID-19. The algorithm was able to adjust to different phases of the pandemic, with four times greater accuracy than random surveillance testing at identifying asymptomatic carriers. Carefully designed AI may not be vulnerable to the same problems that we are currently seeing due to COVID.
Algorithms can improve efficiency in a variety of industries. But the pandemic has provided several examples of AI algorithms going awry without people realizing it. This is a serendipitous opportunity to develop and test ways to reduce vulnerability to similar “shocks” in the future. That way, the next pandemic, economic downturn, or other global disruption won’t incapacitate our algorithms along with it.
You May Also Like
Cracking Open the Black Box (Open Technology Institute, 2021): -- Machine learning and AI have gained significant traction in the past decade, used by private companies and government agencies alike. Unfortunately, these capabilities also have the capacity to create discriminatory and biased outcomes. New mechanisms to promote Fairness, Accountability, and Transparency (FAT) have failed to account for the numerous accountability mechanisms that must simultaneously be developed to promote meaningful FAT. This report explores nine different approaches internet platforms and governments can take to promote fairness, accountability, and transparency in AI.
The Building Blocks of Meaningful AI Regulation (Open Technology Institute, 2021): -- Algorithmic systems are increasingly used in our everyday lives, from home lending to job hiring to ads targeting higher education. These algorithms frequently generate biased outcomes, using historical data that reflects societal biases. Lacking sufficient standards and frameworks to assess high-risk AI systems, we need to come together to think through an approach to reign-in the use of high-risk algorithms.
Regulating Platform Algorithms (Open Technology Institute, 2021): -- The EU has responded to the risks posed by Artificial Intelligence by creating two comprehensive legislative proposals. Comparatively, U.S. policy proposals are harder to enforce with the challenges posed by the First Amendment. This brief outlines five different categories of legislative proposals that both the EU and U.S. policymakers have explored to regulate AI and offers recommendations on how to improve them.
Future Tense is a partnership of Slate, New America, and Arizona State University that examines emerging technologies, public policy, and society.