The Building Blocks of Meaningful AI Regulation
Article In The Thread
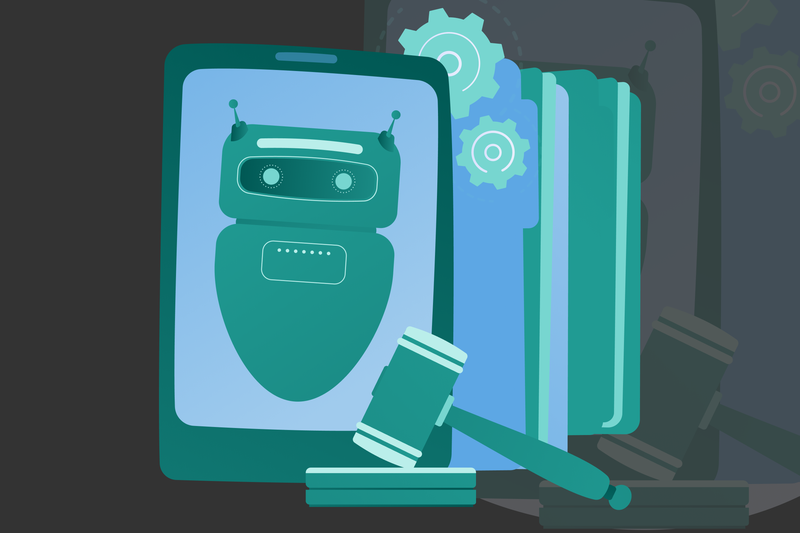
New America / Visual Generation on Shutterstock
Oct. 12, 2021
Buying a home is an important milestone many Americans dream about. Kids grow up doodling images of their dream home. College students start building their credit early so they can apply for a mortgage in the future. People save money for years so they can afford a downpayment. But, imagine if after all that dreaming and hard work, your hopes of buying a home are dashed by a biased lending algorithm that uses your race, or where you grew up, to determine your future.
According to a recent investigation conducted by The Markup, this nightmare is a reality for many prospective borrowers in the United States. The investigation found that in 2019, lenders were more likely to deny home loans to people of color than to white individuals who shared similar financial characteristics. The Markup reporters looked at over 2 million mortgage applications and found that across the United States, lenders were 40 percent more likely to reject Latinx applicants, 50 percent more likely to reject Asian and Pacific Islander applicants, 70 percent more likely to reject Native American applicants, and a shocking 80 percent more likely to reject Black applicants than white applicants with similar financial attributes. Many of these decisions were made by black-box algorithms that generated biased outcomes even when reporters controlled for financial factors the mortgage industry claims account for racial disparities in lending.
Home lending is just one example of a sector that relies on algorithms to make consequential decisions about people’s lives. Governments and companies are increasingly relying on artificial intelligence and machine learning-based tools to determine everything from job hiring to targeted ads for higher education that can unintentionally exclude certain demographics of people. Many of these tools use historical data that reflects societal biases. Algorithms are unable to understand that racism, sexism, homophobia, and other patterns of discrimination are harms that our society has perpetuated and must reckon with, not norms to be replicated and exacerbated. For this reason, many lawmakers and civil society organizations have begun thinking through how we can promote accountability around the use of algorithmic systems that pose a high risk to individuals and their fundamental rights.
Earlier this month, New America’s Open Technology Institute (OTI) released a report that explores nine different approaches internet platforms and governments can take to promote fairness, accountability, and transparency around high-risk AI systems. We also hosted a panel event on this issue. As we continue to think through how to reign in the use of high-risk algorithms, the private sector, government agencies, lawmakers, civil society, and academia need to come together to achieve three critical steps.
Imagine if after all that dreaming and hard work, your hopes of buying a home are dashed by a biased lending algorithm that uses your race, or where you grew up, to determine your future.
First, we need to clearly define high-risk AI. In the EU, lawmakers have proposed a definition in their draft AI regulation. For U.S.-EU regulations to have some degree of harmony, we need consensus around how to define high-risk AI as these policy conversations across the pond begin to percolate in the United States. Clear definitions will make it easier for companies and agencies to comply with guidance and make it easier for civil society, academia, and regulators to provide meaningful oversight.
In addition, we also need greater collaboration on fairness, accountability, and transparency work more broadly. As OTI highlighted in our report, there are many existing approaches for promoting fairness, accountability, and transparency around high-risk AI systems, ranging from transparency reports to machine learning documentation frameworks, to algorithmic audits. However, communities working on these disparate interventions rarely come together. This gap is especially prominent between technical, policy, and legal communities. As a result, there is little dialogue around how these different approaches can supplement existing gaps and work in tandem to create a comprehensive approach to promoting fairness, accountability, and transparency around high-risk AI systems.
Lastly, we need clear guidance for algorithmic evaluations. We currently lack sufficient standards and frameworks to assess high-risk algorithms for fairness, accountability, and transparency. Both EU and U.S. lawmakers have recently begun including approaches such as algorithmic audits and impact assessments in draft legislation. But in order for regulations to be meaningful, we need clear guidance around how these evaluations are being implemented, who is implementing them and why, and what the expectations around transparency and accountability are. Without this guidance, efforts to mandate fairness, accountability, and transparency in high-risk algorithms may falter and subsequently fail to protect the fundamental rights of the communities already most vulnerable to algorithmic bias.
Government agencies and private companies are increasingly using algorithms to make important decisions about people’s lives. While these systems can introduce scale and ease into important decision-making processes, we’ve also seen that they can also perpetuate societal inequities. We need more collaborative efforts to promote fairness, accountability, and transparency around these systems before we can trust them to wield so much influence over our lives.
You May Also Like
Cracking Open the Black Box (Open Technology Institute, 2021): With notable drawbacks to fairness, accountability, and transparency, agencies that rely on algorithmic systems must prioritize meaningful and comprehensible information in order to crack down on the discriminatory biases within these systems.
Trained for Deception: How Artificial Intelligence Fuels Online Disinformation (Open Technology Institute, 2021): Social media platforms rely on AI to engage with users and to amplify popular media — but many of these AI tools promote digital deception, leading to legislative efforts here and abroad to tackle the amplification of misleading online information and initiate accountability.
Automated Intrusion, Systemic Discrimination (Open Technology Institute, 2020): Machine learning tools can have dangerous effects on privacy and civil rights. Institutions must examine their own biases to address the equity and privacy risks that make the benefits of AI seem obsolete and ensure equitable use of MI/AI systems.
Follow The Thread! Subscribe to The Thread monthly newsletter to get the latest in policy, equity, and culture in your inbox the first Tuesday of each month.