What Will It Take to Achieve Truly Data-Driven Policy?
Weekly Article
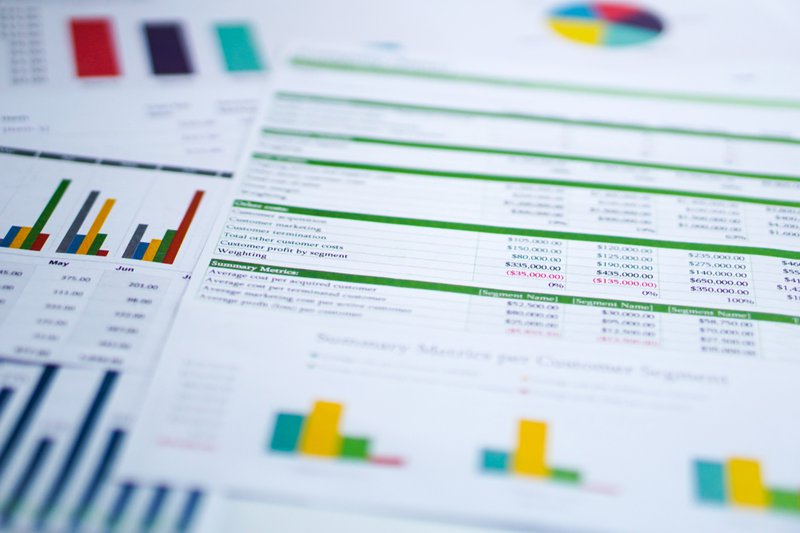
sasirin pamai / Shutterstock.com
Aug. 9, 2018
As the thinking goes, you can’t manage what you can’t measure.
In 2016, the National Academies of Sciences, Engineering, and Medicine was charged with examining the impact of permanent supportive housing programs on health and healthcare costs. In a report released earlier this month, the Academies noted that while this sort of housing likely improves health, there was “no substantial evidence” to prove it. Why? The group concluded “less than it had expected would be possible when embarking on this work” largely due to the absence of relevant data, which either hadn’t been collected or was otherwise unavailable.
And yet, the Academies’ data dilemma isn’t unique. We, as a society, often invest significant resources into ambitious public policies. But despite the time and money we spend doing this, we struggle to determine whether these policies have successfully met their goals. In no small part, that’s because we typically lack monitoring and evaluation mechanisms that can help us decide whether policies are really effective. As a result, failing policies may be left in place, rather than tweaked to reach their intended outcomes. Or, as in the example above, policies that are bringing value may be unable to demonstrate it—and they may then be vulnerable to funding cuts.
Either way, the public loses: Policymakers miss an opportunity to advance good policy, taxpayers don’t see a return on their investment, and those whom a policy is intended to help aren’t served. In other words, it’s hard to make good policy when we don’t know how, why, or when policies are doing what they’re supposed to do. So, how do we achieve truly data-driven policy?
While many policymakers show a growing appetite for evidence-based or data-driven policy, attempts to evaluate policies are often quashed by the very same barriers that beleaguered the National Academies: a lack of data. It may seem somewhat strange, in today’s world of heavy information collection, that we don’t have the data necessary to appraise policy. But that’s at least partly because we often design data-collection forms and processes with operations, rather than evaluation, in mind. As a result, sometimes, the data needed for evaluation isn’t collected at all. And at other times, it’s collected in a way not accessible to researchers.
If policymakers want to reap the benefits of data-centric policy, they must prioritize data and evaluation from the outset. This means deciding what must be measured, and then determining how it will be collected, made available to the public, and analyzed. Luckily, a number of groups are making all this less abstract, as they lay the foundations for more data-driven policy.
Take, for instance, New York City’s Criminal Justice Reform Act (CJRA). The New York City Council designed the CJRA, which allows low-level misdemeanors like violating park rules or drinking from an open container to be diverted from criminal justice system to a civil court, with future evaluation in mind. Crucially, the law’s authors pointed out the CJRA’s potential to reduce racial and geographic disparities among low-level offenses, and passed legislation to ensure that progress toward this goal could be measured. One bill in the CJRA, in particular, requires the city’s police department to publicly report, each quarter, counts of criminal and civil summonses issued by offense, race, and geography, among other factors. Data-wise, the CJRA’s success lies in the trifecta of making clear what needs to be measured, compelling police to collect relevant data, and creating mechanisms to share results. The quarterly reports, along with a larger policy evaluation, will in turn help the council—and the public—see if the CJRA is meeting its goals, and it can inform future policy discussions on how to improve or expand the policy.
So, the CJRA offers an example of how policymakers can lay the groundwork to measure the success of a new policy. But what about when a policy is already in place?
In this instance, policymakers can work retroactively to outline metrics, collect data, and promote analysis of existing policies. Let’s look at California. There, county and state leadership have noted shortcomings in evaluating the impact of CalWORKS, a major cash assistance program funded through federal dollars. According to these stakeholders, data practices comply with federal reporting regulations—but fail to measure whether the program is achieving its goals of improving the lives of recipients. To address this, the California legislature passed legislation mandating a new performance management system for the CalWORKS. As a result, stakeholders will now outline what should be measured to track CalWORK’s success, and in turn they’ll ensure that important, relevant data is collected and scrutinized.
A working group is currently crafting metrics to do just that. By 2019, counties in California will be required to track related data, as well as provide annual progress reports on these metrics. On top of that, every three years, counties will have to conduct their own self-assessments, based on the data, as well as develop an improvement plan to fine-tune these indicators. While it’s most efficient to establish metrics and collect data from the very beginning, California’s efforts to re-evaluate a major welfare program show that it’s never too late to improve.
This isn’t to suggest that we ought to treat data as if it’s infallible. The opportunity for additional governments to follow the highlighted examples is tremendous, but it’s also key to recognize the limitations, even risks, of data analysis. In their report to the legislature, California’s Legislative Analysis Office echoed the potential for better performance management to improve the state’s welfare program, but noted various challenges presented in the analysis and interpretation of data on policy outcomes, such as the risk of over-attributing positive outcomes to a policy when other factors may have played a role. Existing government initiatives provide some ideas of what this sort of forward-thinking precaution can look like. The United Kingdom’s Justice Lab, which evaluates government and non-profit programs, publishes plain language explanations of their analyses that spell out what conclusions can—and can’t—be drawn from the analysis.
Policymakers must also work to protect against potential harms of data analysis. A growing body of research shows that, without careful consideration, this sort of collection can work against the people policymakers intend to help. Cathy O’Neil has noted that while the public often reveres math and statistics as objective, analysis usually still reflects intentional or unintentional biases. In a similar vein, Virginia Eubanks has found that large troves of government data can create algorithms that surveil and punish citizens, especially vulnerable ones. Some policymakers have already started taking steps to ward off the potential dangers of data. For instance, New Zealand’s chief government data steward recently released a set of principles to guide the government’s data collection and use in order to mitigate the potentially negative consequences of data analysis. These principles enshrine a commitment to protect personal information data used in analysis, and to monitor and address potential bias in analysis.
As people from city council members to state legislators continue to prioritize evaluation in their approaches to policy, reports like the Academies’ ought to become relics of the past. Collecting good data is difficult, yes, but that shouldn’t stop us from measuring our policies so that we can unearth best practices—good policy and people’s livelihoods depend on it.