A Look Under the Hood of Student Tests
Blog Post
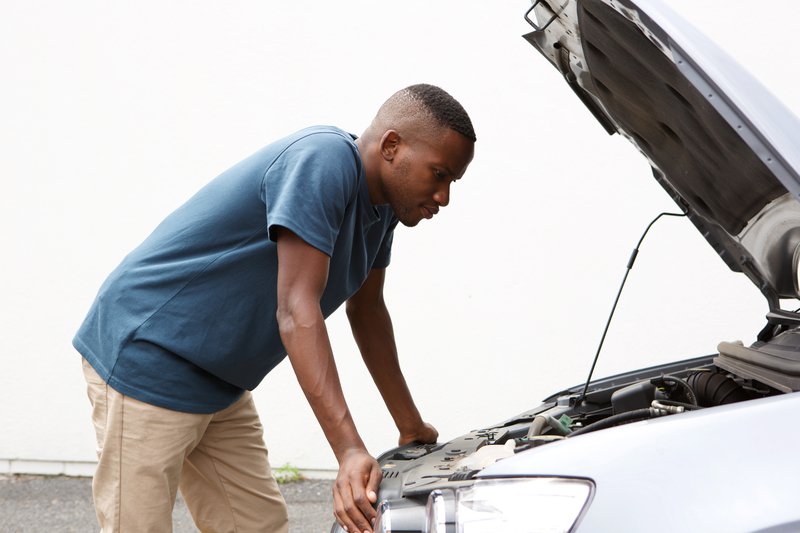
Aug. 29, 2016
For all the concern about the tests our students take in school, there is a central aspect of test quality that the public conversation on testing has largely failed to include: how students’ state test scores are calculated and reported. It’s not hard to imagine why—the process is complex, often confusing, and nearly always explained in dense technical reports that assume a level of expertise that few of us possess. A new report from the Economic Studies program at the Brookings Institution aims to give those of us without that technical knowledge a quick look under the hood of student tests, and makes the case for why policymakers and the public alike should know more about the math and science that drive our students’ assessments.
The report highlights several key choices psychometricians (the scientists who design assessments) make when they design cognitive assessments, and then explains how these choices can impact data and accountability systems. These considerations include the test’s length, whether or not it is computer adaptive, and whether or not students scores are ‘shrunken.’ If the jargon is off-putting, not to fear: author Brian A. Jacob says that experts need to do a better job of fully explaining what these concepts are and why they are important, and attempts to do so in his report.
Amid complaints about perceived over-testing in public schools, state and district administrators (with guidance from the Federal Department of Education) have sought ways to reduce testing time. A large part of this effort is cutting duplicative assessment, but some conversation has also centered on reducing the length of tests. Jacob’s paper argues that, given what psychometricians know about the relationship between a test’s length and its reliability, this could be a mistake. The fewer questions on a test, the more likely that a student would score differently if they took the assessment multiple times, meaning that the test is a less reliable measure of the student’s knowledge and skill. If states want to reduce test time by shortening the actual length of their assessments, this should be an important consideration—in doing so, they could unintentionally dilute the effectiveness of those tests.
The report also touches briefly on several implications of using item response models, which underlie computer adaptive assessments. Computer adaptive assessments differ from traditional tests in that the students answer change based on how they demonstrate understanding on previous questions. In the hopes that they will give a greater depth of information about individual students’ mastery, computer adaptive assessments are being used more and more in states. The Smarter Balanced Assessments, for instance, which were used fourteen states this past year, are all computer adaptive. The systems underlying adaptive assessment are understandably complex, and merit greater explanation than a short report could do justice, but Jacob’s point stands: there is a lot that we don’t understand about how adaptive assessments work. If policymakers are using data from a computer adaptive test to make decisions, they should understand how that test is constructed.
Just as important as how a test is built is how its data is analyzed and reported. Jacob notes one procedure that he says is common in processing test score data, which results in ‘shrunken scores.’ In Jacob’s words, this means that “instead of simply reporting a student’s score based on the items that he or she correctly answered, the test developer reports what can be thought of us a weighted average of the student’s own score and the average score in the population.” This controls for the level of measurement error that is present in any assessment. The technique affects the most extreme scores (highest and lowest), assuming that in both cases, some amount of chance was involved in students scoring extremely high or very low.
Shrinking scores, then, moves a higher proportion of students out of the highest and lowest achievement brackets and pushes them toward the mean. When scores are shrunken, then, we should see a higher proportion of students generally scoring around proficient, with fewer scoring far below and above proficiency. Of note, shrunken scores may lead observers to underestimate differences between student subgroups, such as the proficiency gap between black and white students, because students in the lower-performing subgroup (in this example, black students) will, on average, have their scores adjusted upwards toward the mean, while those in the higher-performing subgroup (white students) will have their scores adjusted downward towards the mean. Jacob does not list which state K-12 assessments shrink scores, nor how significantly the process impacts student proficiency rates. Given that so much rides on proficiency data, however, he makes the case that policymakers should at the very least understand how it could affect the information they receive back from test vendors.
The report touches on several other test construction and score reporting techniques that could impact students’ scores. Though Jacob doesn’t give an exhaustive look at any of these techniques, he calls attention to a whole host of considerations that many with a stake in K-12 tests don’t actually know or understand about them. There must, he says “be a greater transparency about how test scores are generated. Researchers, policy analysts and the public need to better understand the tradeoffs embedded in various decisions underlying test scores.”
Armed with a more complete understanding of how tests are designed, policymakers can make better-informed choices about how to select the right assessment of student learning, accurately interpret its results and ultimately, act on test data to help students achieve more.