Open (AI) Skies
Leveraging Emerging Technologies to Improve International Transparency and Stability
Brief
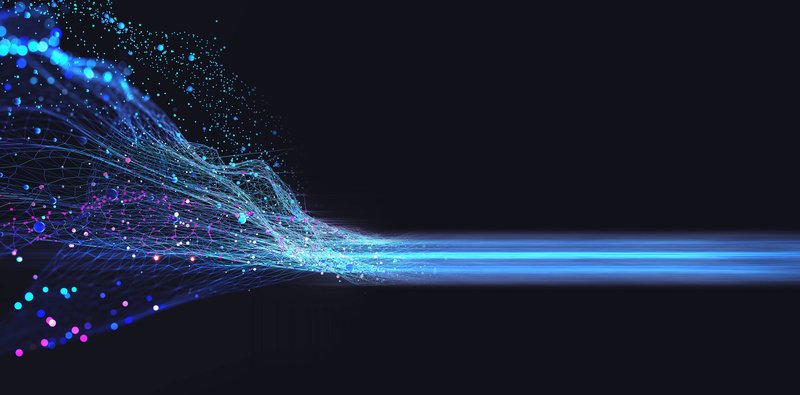
Shutterstock
Oct. 10, 2023
This brief is part of a series by New America’s Nuclear Futures Working Group, which brings together emerging researchers from academic, government, advocacy, and policy spaces to develop research on nuclear security policy problems through the lens of a changing global environment.
Executive Summary
Intelligence, Surveillance, and Reconnaissance (ISR) capabilities that leverage artificial intelligence (AI) are making it possible for decision-makers to derive meaning from vast amounts of data collected by disparate sensors. While this technological development improves strategic stability by enhancing situational awareness, it also risks creating new pathways for unintentional conflict escalation—including escalation to the level of nuclear use. Recommitting to the principle of mutual transparency could preserve the benefits of employing machine learning to process ISR data while minimizing the risk of unintentionally exacerbating tensions and conflicts.
Many technologists, activists, and scholars have emphasized diplomatic approaches to the banning of AI-enabled military capabilities that circumvent human decision-making. Relatively less attention has been paid to opportunities to collaboratively leverage the benefits created by AI while working to minimize the very risks it may exacerbate. The Open Skies Treaty (OST) is an instructive model for future cooperative efforts to reduce the escalation risks posed by remote monitoring tools that leverage machine learning. A new international agreement based on OST would establish a network of sensors, including satellites and unmanned aerial vehicles, and collectively develop transparent algorithms to process the data they collect. This approach would create a legitimate and shared avenue for applying AI to ISR, reduce incentives for governments to leverage AI applications that might carry a greater risk of escalation, and promote dialogue in periods of crisis by creating sharable collateral.
Policy Recommendations:
- Negotiate a treaty based on the mutual transparency framework of the Open Skies Treaty to leverage advances in machine learning and contribute to a more stable international security environment.
- Participating governments should collectively establish a network of sensors that is distinct from any single state’s sensing capabilities to improve trust and create redundancies.
- Develop an international cohort of machine learning experts from participating states to create transparent, collectively owned algorithms to process data collected by the shared sensor network.
- Continuously share raw data and machine learning insights with all participating states.
The Problem: AI, ISR, and Escalation
Scholar James Johnson has noted that “Even if [AI-augmented] Autonomous Weapon Systems (AWSs) are only used for conventional operations, their proliferation could nonetheless have destabilizing implications and increase the risk of inadvertent nuclear escalation.” The incorporation of artificial intelligence (AI) into Intelligence, Surveillance, and Reconnaissance (ISR) systems exacerbates existing escalation risks among nuclear-armed states in three ways: by creating new single points of vulnerability; by blurring the boundary between conventional and nuclear capabilities; and by incentivizing rapid action before the value of an insight generated by machine learning expires.
Escalation Pathway 1: Single Points of Failure
The U.S. Department of Defense’s Third Offset Strategy, a guiding principle of defense planning and acquisition since the 2010s, emphasizes robotics, autonomy, and connectedness among weapons systems. It envisions a future of warfare where sensors like radar systems and global imaging satellites communicate seamlessly. This strategic concept has advanced significant investment in cyber-enabled platforms. Acquisition strategies that prioritize the development of a small number of platforms that communicate with and control many capabilities create single points of failure: An attack targeting a single platform could result in the failure of the entire network of systems. Capabilities to target, damage, and disable digitally enabled sensor platforms; command, control, and communications capabilities; and weapon systems have developed more quickly than the Department of Defense (DOD) has been able to take full advantage of.
While there are many advantages to integrating machine learning in defense systems, the benefits are accompanied by significant risks. The vulnerability of artificial intelligence to cyberattacks means that adversaries can threaten networks of U.S. capabilities without necessarily using conventional weapons or causing physical destruction.
U.S. military operations rely on a relatively small number of systems that contain many sensors and depend on digitally sharing information with other platforms. The cost of these advanced, highly connected systems precludes the DOD from establishing redundancies and backups in case they are disabled. In part because of this, adversaries may be incentivized to strike the infrastructure that supports U.S. weapon systems, including intelligence processing centers and communication satellites, to disable these capabilities. An attacker might strike opportunistically or if they believe that the United States is preparing to attack.
Alternatively, U.S. military planners and decision-makers may be incentivized to use the country’s expensive, connected systems to conduct a first strike early in a crisis or conflict before those capabilities can be disabled by an adversary if they perceive that critical capabilities might be targeted. The Hoover Institution’s Jacquelyn Schneider refers to this dynamic as a “capability/vulnerability paradox.”
Escalation Pathway 2: Blurred Boundaries
The boundary between conventional and nuclear systems and missions is becoming increasingly blurred. Systems that support conventional missions—which include satellites that serve command and control and ISR purposes and certain dual-use delivery systems—can also be used to support nuclear missions. Known as “entanglement,” this dynamic exacerbates the challenge of single points of failure. For example, an actor seeking to degrade U.S. conventional warfighting capabilities via a direct-ascent antisatellite weapon may unintentionally threaten U.S. nuclear forces, leading decision-makers in Washington to employ those forces before the capability, or the ability to employ that capability, is destroyed.
Similarly, ISR operations intended to gather data about a country’s conventional forces may intentionally or unintentionally reveal information about its strategic forces. States that no longer perceive mobility and concealment as effective means of securing a second-strike capability may feel that they must use the destructive capabilities in their possession before they are neutralized. One report published by the Center for Strategic and International Studies argues that ISR capabilities that are intrusive (they enter another state’s airspace without permission), or vulnerable (they are susceptible to an incoming attack), may be especially likely to cause escalation. Technologies such as crewed and uncrewed aerial vehicles that are not stealthy and must enter into another country’s airspace to perform their surveillance missions may be especially likely to cause an escalation.
ISR capabilities that are intrusive or vulnerable such as unmanned aerial vehicles (UAVs) may be especially likely to trigger escalation in a crisis as the country being observed is likely to notice an intrusion into its airspace. According to a recent funding solicitation from U.S. Special Operations Command, machine learning could allow UAVs conducting ISR missions to “generate maps rather than rely on them.” While this would improve U.S. capability by reducing reliance on space-based global positioning systems that could be disabled in the course of a conflict, this application of AI could inadvertently escalate a crisis or conflict if a strategic competitor believes that it could empower the United States to carry out a massive counterforce operation that undermines its secure second-strike capability.
In the summer of 2019, Iran shot down an RQ-4A Global Hawk surveillance UAV over the Strait of Hormuz. The incident took place amid already strained tensions between the United States and Iran after the United States accused Tehran of attacking two oil tankers. Then-President Donald Trump stoked fears that Washington would retaliate when he posted on social media that “Iran made a very big mistake!” While this incident did not cause further military escalation, it illustrates how a surveillance asset’s relative intrusiveness and vulnerability may impact the likelihood of escalation.
A report from the Stockholm Peace Research Institute notes that “[a]dvances in AI reinforce the problem of entanglement between nuclear and conventional systems” in part because they contribute to the development of more accurate, precise conventional weapons that are more difficult to defend against and are more likely to credibly threaten nuclear forces.
Escalation Pathway 3: Perishable Insights
Third, in the context of a crisis or a conflict, machine learning algorithms are especially well suited to generating time-sensitive insights. All ISR insights are based on constantly changing information and only maintain their value for a period of time, but machine learning algorithms can comb through vast amounts of data and identify connections among data points that are not likely to be identified by human analysts. As machine learning is increasingly integrated into ISR processes, decision-makers will face both a growing number of opportunities that may not have been identifiable before and a growing number of risks.
For example, a U.S. decision-maker considering information that has been processed by an algorithm may want to take immediate action based on that information rather than miss out on an opportunity. If machine learning supports the identification of a mobile missile such as one of Russia’s 9M729 intermediate-range missiles, which are nuclear-capable, U.S. military leaders might be incentivized to conduct a preventive or preemptive strike to neutralize the potential threat. However, even if an algorithm makes it possible to locate a specific asset, it will not be able to confirm whether a missile poses an imminent threat. Due to confirmation bias, decision-makers could choose to strike a target to prevent a crisis from spiraling out of control.
Related to this risk is the possibility that adversaries may perceive that U.S. leaders have greater opportunities to strike valuable assets and that they are likely to act on those opportunities. Adversaries could hold this perception even if the United States lacked the functional capability or intent to act on machine learning-enabled insights. The belief that assets previously considered to be secure—whether because they are mobile and difficult to track, concealed and difficult to find, or hardened and difficult to destroy—are newly vulnerable could cause adversaries to use those assets prematurely. This could escalate a crisis or conflict that might otherwise have been resolved through diplomatic means.
How Algorithms Detect
While algorithms that support conventional and strategic ISR missions are likely classified by the U.S. government and proprietary to the contractors that develop them, it is possible to speculate about how they might function. An algorithm may share characteristics with the compound algorithms used by companies like Waymo to give self-driving cars the ability to navigate using computer vision. One part of the algorithm would function by unsupervised learning, clustering visual data that is collected, such as a video feed, based on many different factors. In a self-driving car, this might be used to determine whether a pixel in its line of sight is part of the road and therefore safe to drive on or the side of the road, which should be avoided. An unmanned aerial vehicle tasked with conducting surveillance could use a similar unsupervised learning algorithm to categorize objects and identify potential targets.
Deep neural networks (DNN) could enhance this type of algorithm. Tunable connection strengths allow for DNNs to “strengthen certain pathways for right answers and weaken the connections for wrong answers.” One characteristic of DNNs is that they have many layers between input and output layers. According to expert Paul Scharre, “extra layers allow for more variability in the strengths of different pathways and thus help the AI cope with a wider variety of circumstances.” In the context of hypersonic missile development, neural networks may be capable of learning “underlying nonlinear relationships between the state and optimal actions for nonlinear control problems.”
While deep learning networks can be powerful tools for solving problems and drawing connections among data that are not necessarily evident to humans, their function is not fully understood even by the engineers who build them. This “black box” effect poses accountability challenges in venues ranging from medicine to warfare. In the case of ISR capabilities, operators may be incentivized to act on insights that cannot be fully explained. To be certain, human decision-making is similarly opaque, but where humans can at least attempt to answer questions and can be influenced by negative consequences, including punishment for poor or negligent decisions, algorithms cannot.
The Diplomatic State of Play
Even during the most tense periods of the Cold War, Soviet and U.S. leaders shared the view that a degree of mutual transparency is key to preventing unintentional escalation. Unfortunately, many of the legally and politically binding agreements intended to foster the exchange of information during this period such as the Helsinki Accords and the Treaty on Conventional Forces in Europe have suffered from dysfunction. Post-Cold War treaties that included transparency measures regarding strategic nuclear weapons were a key component of U.S.-Russia efforts to safeguard strategic stability. These included data exchanges, sharing of telemetry data, and on-site inspections. Amid tensions over Russia’s invasion of Ukraine, Moscow “suspended” its participation in the New Strategic Arms Reduction Treaty (New START) in February of 2023.
The Open Skies Treaty, which forms the basis for the policy proposed in this brief, is similarly defunct. The purpose of this 2002 agreement was to reduce the risk of military escalation by allowing states to monitor one another’s military forces and activities. Citing Russian violations of the agreement, the United States withdrew from Open Skies in 2020. The Russian Federation and Belarus likewise withdrew in 2021.
Diplomatic Initiatives on AI
While arms control agreements aimed at strengthening strategic stability are faltering, international efforts to govern the use of machine learning algorithms are in their early stages of development and negotiation. On the use of machine learning in the defense sector, a coalition of nonprofit organizations called the Campaign to Stop Killer Robots is encouraging governments to forego developing and acquiring autonomous weapon systems that exclude human decision-making in favor of systems that maintain a “human in the loop.” This campaign is ongoing but lacks a treaty vehicle in the United Nations system.
Beyond the defense sphere, the European Commission is advancing a set of ethical principles for trustworthy AI. This framework is a rights-based approach that includes human agency, resilience to cyber attacks, privacy and data security, and transparency. While these guidelines are a positive step in developing AI governance structures, they are voluntary and unenforceable.
Despite the challenges of establishing international governance frameworks around machine learning, especially in the sensitive defense sector, it is clear that there is a public and political demand to mitigate the foreseeable risks of AI algorithms while capitalizing on their potential benefits across a range of professional fields. By focusing on collectively reaping the benefits of machine learning in international security, an AI-enabled update to the Open Skies Treaty could help policymakers meet this growing demand.
Recommendation: Update the Open Skies Treaty for Today’s Challenges
The core mechanism of the 2002 Open Skies Treaty is the principle of mutual transparency. When the treaty was proposed and negotiated, creating a pathway for states to overfly a potential adversary or competitor’s territory was intended to reduce the risk of inadvertent escalation by improving predictability. Confidence-building and information-sharing among member states are enabling conditions of this agreement. Images captured during overflights are shared among all state parties, even if they did not directly request the overflight. This allows governments to address one another’s military activities without divulging sources and methods of intelligence collection. It also minimizes plausible deniability for states that might seek to carry out malicious acts while avoiding attribution and accountability. Short-notice overflights can decrease uncertainties among states and assure governments that a rival state is not preparing a surprise attack. Additionally, creating a straightforward mechanism for states to gather information can reduce the incentives for governments to employ intrusive or vulnerable methods that can ultimately cause escalation even if that is not their purpose.
Despite the challenges encountered by diplomatic initiatives seeking to improve communication and information-sharing about military capabilities, the principle of mutual transparency remains valuable in preventing unintentional escalation. Depending on how states choose to negotiate a new agreement that combines the underlying logic of the original Open Skies Treaty with advances in machine learning, participating governments could establish a fleet of shared ISR assets including satellites, UAVs, or both. Countries already rely on these tools for intelligence gathering, just as aerial overflights by manned aircraft were in wide use when the Open Skies Treaty was concluded. One key difference between aerial overflights and an expanded slate of ISR capabilities that includes satellites is the persistence offered by space-based capabilities. A machine learning algorithm developed cooperatively and transparently by member states could assist with processing the vast amount of data gathered by shared satellites and more intrusive ISR capabilities like UAVs.
In parallel to the Open Skies Treaty, a similar mutual transparency mechanism for AI-enabled ISR capabilities could particularly benefit small states that do not have advanced satellite monitoring capabilities. The information-sharing aspect of the original Open Skies treaty allowed small states to access information that they might not have had access to otherwise.
Proprietary concerns regarding technology ownership are likely to pose an obstacle in executing this approach. Some countries that possess highly developed AI sectors—including the expertise, access to data, and economic backing—may be hesitant to share their gains with states that have less AI experience. Countries that compete with the United States, specifically China, could elect not to engage with a future agreement or treaty, preferring to maintain its purported AI advantage. Even in the United States, the large private technology companies that dominate AI development and implementation may have concerns about sharing proprietary algorithms and datasets. For these reasons, at least early on, machine learning tools developed through this treaty framework would likely need to be relatively rudimentary to reassure states and private companies that participation would not compromise proprietary information.
Thomas Mahnken and Grace Kim have argued that the United States should work with NATO allies to adopt a strategy of “deterrence by detection,” leveraging persistent surveillance capabilities to predict and preempt opportunistic aggression. Such a strategy would undoubtedly be considered provocative and escalatory by strategic competitors like Russia and China, but a cooperative agreement to leverage ISR capabilities and machine learning algorithms to derive insights from surveillance data could provide some of the same benefits. A diplomatic agreement to develop shared machine learning capabilities in service of mutual transparency could deter states from planning and executing attacks by creating a common record of sharable surveillance data and limiting plausible deniability. It would also serve the Open Skies Treaty’s original objective of reducing military uncertainty among states.
Because an approach modeled on the Open Skies Treaty hinges on permitting defined activities within a specific range of parameters, it would not prohibit national governments from adopting AI in other functions that could enhance deterrence. In contrast to an approach that seeks to ban certain military applications of AI, this pathway might be more politically viable in countries where machine learning holds the most promise for defense purposes. Like Open Skies, this type of confidence-building measure would be most effective if it were developed alongside diplomatic agreements modeled on treaties that prohibit certain particularly destabilizing activities.
Drawing inspiration from disarmament agreements that seek to ban a category of weapons, such as the Chemical Weapons Convention or the Biological Weapons Convention, a companion agreement could ban a narrow set of military applications of AI. Just as biological weapons have historically been considered a tool of weak or belligerent states, technologically advanced capabilities, such as AI-enabled ISR, bestow an element of prestige on national governments and their populations. Imposing an outright ban on integrating machine learning is therefore politically unlikely, but it is also unnecessary to achieve the aim of limiting escalation risks. Yet, restricting the use of AI applications that may exacerbate escalation dynamics could be effective. Another option would be to bar the employment of algorithms that cannot be explained, such as deep neural networks. As research efforts to develop explainable AI (XAI) advance, including those undertaken by the U.S. Defense Advanced Research Projects Agency, additional types of algorithms could be approved for use.
Conclusion
A mutual transparency agreement that leverages AI to draw insights from ISR data may be aspirational in the current geopolitical environment. Nonetheless, diplomatic efforts toward this end are worthwhile. As machine learning advances more rapidly than policies to regulate its applications, opportunities to positively and cooperatively set standards for acceptable use are as important as efforts to restrict the development of certain applications. Despite an unpromising outlook for the Open Skies Treaty, combining the mutual transparency provided by the original agreement with contemporary technologies could enhance international stability and energize diplomatic initiatives on machine learning and other novel technologies.